The disruptions of 2020 elevated the importance of having the right data and insights to pivot quickly when necessary. Here’s a look at how businesses can use embedded intelligence to prepare for uncertainty and meet ever-changing customer expectations.
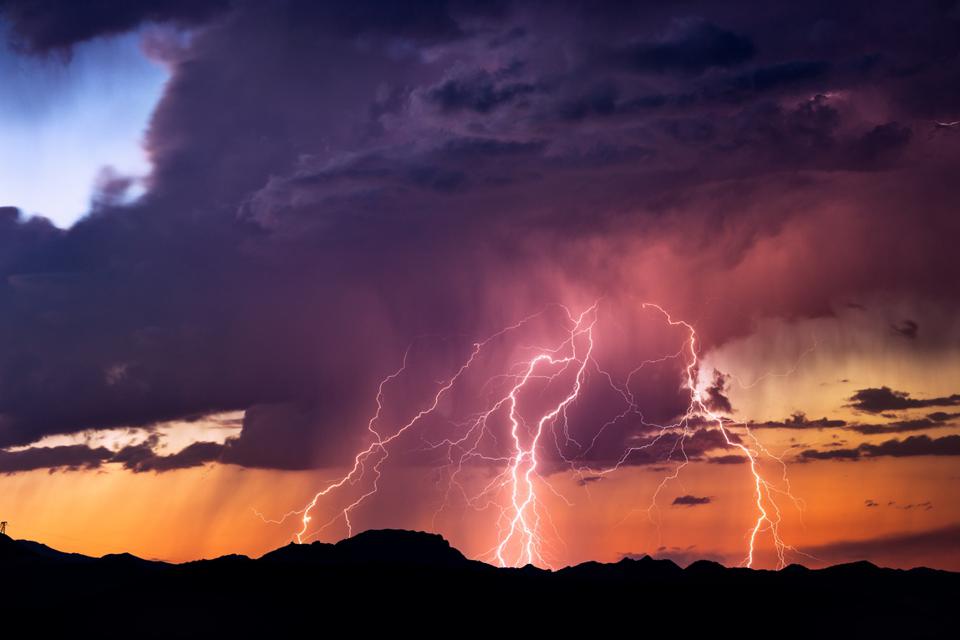
We can never predict the future—but we can prepare for unpredictability by having the agility to always improve, and positioning ourselves to make quick, intelligent pivots when the time comes.
getty
At work and in life, some unpredictability is always a part of the package. Ten years ago, your business might have experienced sudden product demand or a system outage that slowed down deliveries. Servers or data platforms might have run out of capacity earlier than expected.
In 2020, though, the concept of unpredictability in business reached new heights. These disruptions have elevated the importance of embedded intelligence—that is, having machine learning built into the tools people use every day, so that when a pivot is necessary, everyone has the data and insights they need at their fingertips. In fact, 2020 was so disruptive, with so many changes in customer behavior and so many ripple effects, a lot of historical data and forecasting assumptions may not be helpful in 2021. This only increases the onus on businesses to make the freshest data actionable for more of the workforce.
A decade or so ago, the idea of embedded intelligence might have seemed like science fiction. You might remember hearing that analytics would be able to make predictions, and that technology would be able to take on the complex work of predicting retail demand or helping to create a responsive supply chain. But insufficient hardware, older architecture models, slow queries, and untrustworthy data often got in the way.
Now, that concept has become reality as enterprise decision-making has moved from legacy tools to cloud-powered data intelligence services. Today, it’s possible to perform complex analytics tasks and obtain valuable, trusted outputs much faster than ever before. That speed and scale has allowed businesses to tackle entirely new projects and release new features and products very quickly. In addition, APIs have become a lot more intelligent, making it easy to connect siloed solutions. No matter the industry, businesses can access the technology to get to the bottom of what customers need.
Related: Top 5 trends for API-powered digital transformation in 2021
Meeting ever-changing customer expectations with embedded ML
Bringing embedded analytics to real-world uses continues to evolve, with a number of inspiring examples surfacing in the past year. As a result of the pandemic and shifting public health guidelines, many businesses didn’t know month by month if they’d be interacting with customers primarily through in-person or digital channels. And even if both channels were available, it wasn’t obvious how changing customer behaviors would net out.
At patient engagement platform Force Therapeutics, for example, daily activity on their virtual care platform went up by over 140% during the pandemic. With such a large influx of incoming data, it would have been difficult—if not impossible—for a team of humans to gather, organize, and draw insights from all of that information, especially in a timely enough manner to be of use to healthcare providers.
To deliver the necessary care when and how it was needed, Force Therapeutics required a machine learning solution that could identify patient needs based on a wide range of data. Using an embedded analytics platform, they created an application that allowed them to monitor the progress of post-op patients, answer questions, or triage concerns remotely. The platform also enabled providers to check for spikes and anomalies, in order to identify patients who needed to come in due to a critical issue.
Amidst all of the disruption, it became clear that teamwork is essential, and that effective teamwork relies on having the right data-driven tools to get the job done.
Likewise, home delivery became a bigger part of consumers’ routines. This increased pressure on companies to adapt quickly to changes that might prevent packages from arriving on time, such as worsening weather conditions or upstream supply chain disruptions. Amidst all of the disruption, it became clear that teamwork is essential, and that effective teamwork relies on having the right data-driven tools to get the job done.
One example of this can be seen in Google Cloud customers who are using public data to accelerate their journey from data to actionable insights. Some retailers are utilizing the Google Cloud Public Datasets Program to leverage NOAA’s Global Surface Summary of the Day (GSOD) and Severe Weather Data Inventory datasets in order to better understand disruptive weather events, reroute their supply chains to prevent disruptions, and predict their in-store inventory needs to support communities as they recover from natural disasters.
Implementing ML without the complexity
The idea of embedded ML has been hyped for years, but for many use cases, the status quo tools have not caught up to the enthusiasm. Many business intelligence tools rooted in older database architectures require intense engineering work to deliver insights, queries are often slow, and the output is not always consistent or accurate. Part of the challenge is that building ML pipelines is difficult. Data in a database or data warehouse typically needs to move to an intelligence platform so models can be trained, and the models then need to be deployed and integrated into business workflows.
But modern data warehouses such as BigQuery let users train models in the warehouse itself, without having to move the data—and once the models are created, they can be applied and integrated into business processes using simple SQL. When it comes to embedding ML into enterprise processes, these modern approaches significantly lower the barrier for entry. Plus, tools like Looker, Google Cloud’s platform for modern BI and data applications, were created specifically for modern data needs, with the assumption that data needs would constantly evolve and that iterations should be made quickly without eating up inordinate engineering resources.
Related: Google (Looker) recognized in the Gartner 2021 Magic Quadrant for Analytics and Business Intelligence Platforms.
For Commonwealth Care Alliance (CCA), Looker was originally implemented to alleviate their pain points around data bottlenecks and data chaos. But when the pandemic hit, the nonprofit, community-based healthcare organization pivoted to make use of Looker’s tools to better serve patients. CCA used BigQuery and Looker to combine numerous datasources, create a predictive model that assesses risk, and distribute that model to its clinicians. This has given response teams the insights to determine who is too high risk to come in for care so they can reach out with home care solutions.
This kind of agility is not a one-time antidote to a one-time disruption, but rather the norm to which organizations must aspire if they want to remain competitive and protect themselves against future disruptions.
This same functionality is also helping businesses like SoundCommerce. Retailers like Constellation Brands, Eddie Bauer, and FTD/ProFlowers use SoundCommerce’s out-of-the-box data platform, which is powered by BigQuery and Looker, to collect retail data from any source and build a model around the metrics and relationships that are most crucial to retail. This has saved brands hundreds of manual reporting hours each month, and reduced platform licensing costs by almost 75%. Just as importantly, during the uncertain times of 2020, brands that used SoundCommerce were able to align real-time and predictive business decisions across marketing and operations with critical retail KPIs like contribution margin and customer lifetime value (CLV).
As 2020 showed us, we can never predict the future—but we can prepare for unpredictability by having the agility to always improve, and by positioning ourselves to make quick, intelligent pivots when the time comes. Last year was in many ways a rubicon: This kind of agility is not a one-time antidote to a one-time disruption, but rather the norm to which organizations must aspire if they want to remain competitive and protect themselves against future disruptions.
Looking for an ‘easy button’ to speed up your BI workloads running on BigQuery? Check out our latest announcement about BI Engine on the Google Cloud Blog.