Olly Downs, VP of MarTech, Data & ML at Zulily, explains how the online retailer is using the latest tools in artificial intelligence, machine learning, and cloud computing to innovate and serve its customers with purpose.
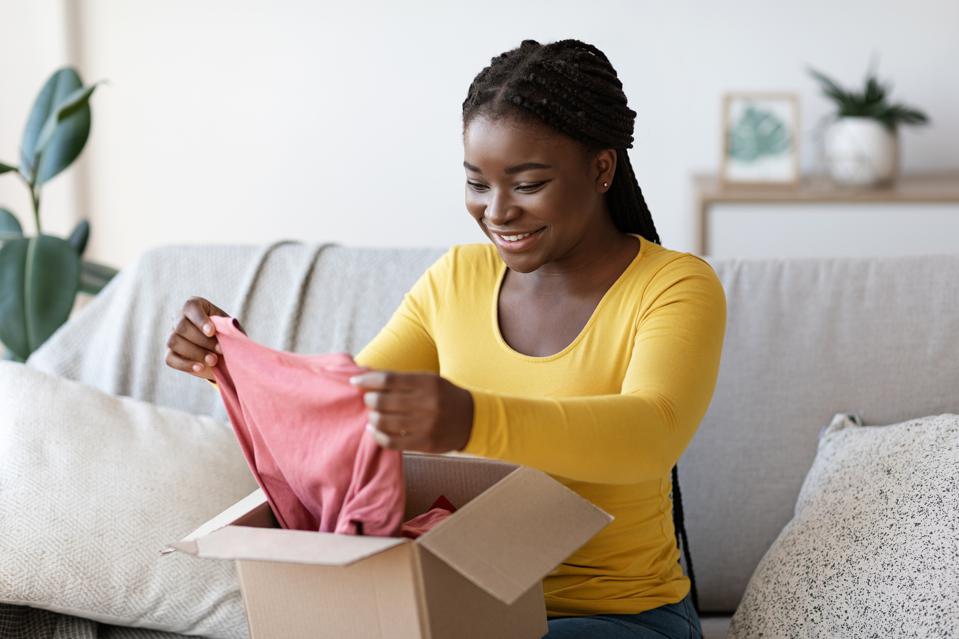
At Zulily, we’re not just continually offering customers the choices we think they’ll like; we’re managing a highly complex and ever-changing supply chain and logistics model, using advanced data analytics.
getty
Living in a technology revolution can sometimes feel like everything has changed. For businesses, technology has changed how we source goods, reach customers, and work with each other. The most critical elements of success, however, remain deeply human.
As both a scientist and technologist, my work is dedicated to serving people and their needs. In an industry like retail, this work consistently adheres to three well-known standards: 1) the customer wants to be understood; 2) markets are based on supply and demand; and 3) do the hard jobs first, and the easy ones take care of themselves.
In the current tech revolution, each of these traditional standards is addressed in new ways. In the case of online retailer Zulily, we have established a set of “new basics” as our north star for business success. Here’s how we see things:
1. The customer wants to be understood
Table of Contents
For most of commercial history, shopping was a pretty personal affair. Small-town store owners intuitively knew the habits of their customers—what kinds of items they liked to buy, what their family’s interests and preferences were, or when a special occasion was coming up. Ideally, “the customer is always right” meant customers felt understood and satisfied.
Then came department stores and mass manufacturing. These offered more selection and better prices, but created a gap between merchants and customers. Direct marketing attempted to close some of the gap with tailored offerings, but infrequently moved past using broad demographic data. Customers rarely felt well understood.
Each of these new basics remain in service of the greatest classic basic: Serve human needs. If technology can help you do that better, use it. If not, question why you’ve got it.
Enter modern retail and ecommerce, with customers on smartphones and retailers crunching vast quantities of data in the cloud. At Zulily, we try to bring that 1:1, small-town store experience to our millions of customers around the world—even as we launch thousands of new products, process billions of clicks from online shoppers, and serve up millions of distinct views into our store via our app and site every day. We target shoppers with offers and products that we predict they will be interested in, and constantly explore new ways to understand them better via rapid experimentation and continuous model improvement.
For example, customers shopping online must have a complete view, beyond product images, of what a piece of apparel will look like when tried on. They want to know the length of sleeves on a shirt or the height of a shoe heel—details missing from most online clothing descriptions. To provide this, we train machine learning models to inspect product images and discern the information. It has become much easier to build solutions like this with the help of cloud-based technologies such as AutoML Vision, and it gives customers the ability to browse and buy more confidently.
Related: Retailers find flexible demand forecasting models in BigQuery ML
2. Markets are based on supply and demand
Whatever the market, there have always been a couple of basic questions: What is there a lot of, and how can we sell it at a fair price? What is hard to find that people want to pay for? How do we judge desire? Supply used to mean raw materials, agriculture, or finished goods. Today, choice is abundant, and it has to be managed. What’s scarce? Time, and the ability to choose well.
Smart companies never lose sight of these basic questions. In the same way that Google refines an abundance of web pages to meet an individual’s needs and intent in a search, Zulily adds 9,000+ products to our online store each morning—a scale of virtual inventory beyond what you’ll find in the warehouses of many retailers.
We “refine” that amount from an even bigger number of possible choices, then refine it further by matching products to likely customers—often under time-limited sales at up to 70% off MSRP. Familiar customers know they have to act fast. We are, in a sense, imposing scarcity while adding a level of engagement and discovery. The experience is meant to be fun and engaging, as merchandise ranges from matching family pajamas to special simmer sauces to trending toys.
We’re not just continually offering customers the choices we think they’ll like; we’re managing a highly complex and ever-changing supply chain and logistics model, using advanced data analytics. Even while we bring in new stock, we measure and predict how fast we can run out of it. Cloud computing helps here too, since it’s easy to scale compute and storage up and down, orchestrate that programmatically, and manage and manipulate our large datasets.
Related: Get research insights and tips to add resilience in retail. Download report
3. Do the hard jobs first, and the easy ones take care of themselves
If you do a lot of work online, you probably have a lot of data. But what does it mean? Does everyone in your organization see your information the same way, and can they all act on it? Is it easy to access and to measure? Data awareness is the hard job.
Obviously, we believe strongly in data analytics and ML to power our business. Even before we use those tools, however, we focus on how we tag our data, and how helpful those tags are in describing things that matter to customers—such as shirt sleeve length or cuff style. On the business end, tags on financial information, like when a sale has closed, matter to the finance and sales departments. In every case, people who have a stake in the data should know and agree on both a data tag and what it represents. That is a slow process, but it makes everything else move faster.
At Zulily, we always strive as data scientists and engineers to embark on any experiment with the three D’s in mind: all of our work must be documented, defensible, and democratized.
Another hard data job is managing the culture of data experimentation. Compared with software engineering, which is based around managing and analyzing data, experiments like changes in page layout or new types of discounts are far less predictable. Data science is about testing ideas, unpredictability, and the necessary failures that accompany most learning. This can upset organizations that aren’t prepared to execute it properly.
The solution is to design experiments to gather as many insights as quickly as possible. In designing these, you can either affect a large portion of the customer experience for a short time, or a small proportion of the customer experiences for a longer time. Once the experiment has concluded, be prepared to act quickly and make changes based on the new insights.
At Zulily, we always strive as data scientists and engineers to embark on any experiment with the three D’s in mind: all of our work must be documented, defensible, and democratized. That means that we can truly ensure that technology and business are in support of one another, and insights are available to our partners within the organization—not just other engineers.
Each of these new basics remain in service of the greatest classic basic: Serve human needs. If technology can help you do that better, use it. If not, question why you’ve got it. We believe in AI, ML, cloud computing, mobility, new delivery mechanisms—all of the cutting-edge tools. We believe there’s lots more to come. But we’re succeeding because we’re concentrating on the essential truths of retail—serving our customers with purpose, and as individuals—and that won’t change in the digital era.
Read this next: Beyond COVID-19, retail looks to transform with AI/ML