Operationalizing and scaling machine learning to drive business value is really hard. Here’s why it doesn’t need to be.
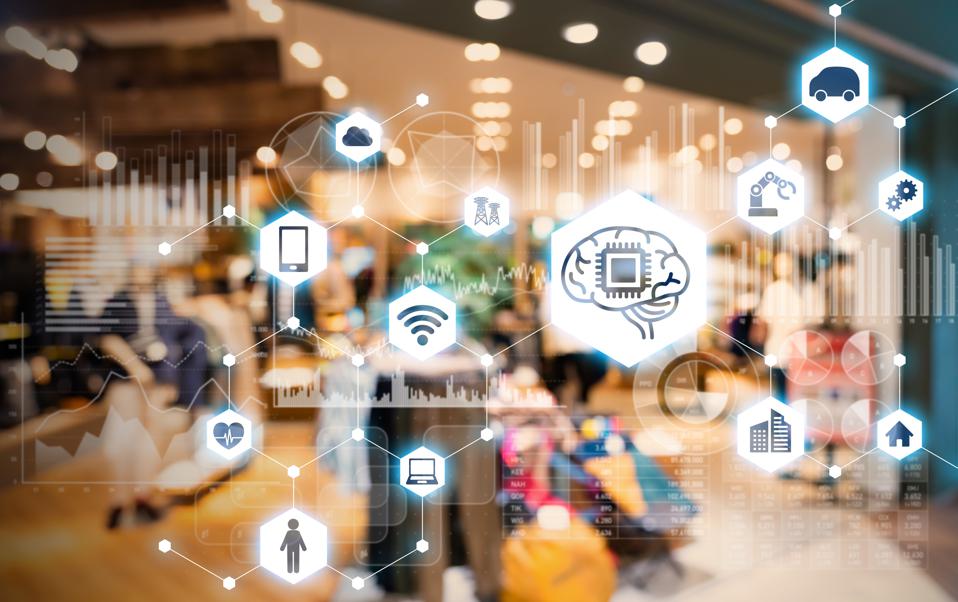
A significant portion of machine learning development has moved to the cloud. MLOps allows businesses to efficiently build and manage machine learning projects throughout the development lifecycle.
getty
It used to be that training machine learning models—the “brains” of artificial intelligence (AI) systems that do everything from serving Google search results to targeting audiences with movies to turning people into cats for their court hearings—took a team of PhDs to get right. It’s astounding to see how quickly this has changed from the domain of experts to literal child’s play—or, at least, something a motivated techie can teach themselves online. Which means more and more enterprises are able to use machine learning to automate, predict, plan, and personalize their products and services.
Indeed, today it’s easy to learn enough about machine learning (ML) and AI to be dangerous. But it’s also, unfortunately, easy to be dangerous. That’s because, while actually building models has gotten easier, translating them from science projects into reliable, scalable software that brings businesses value is still hard.
Consider: retailers often build machine learning models to forecast demand so they know how much product to stock. These models learn buying patterns from a company’s historical sales data, combined with in-house, expert intuition. When done right, they can save millions of dollars on would-be unsold merchandise. But neither data nor expert intuition could predict the sales cycle that started in March 2020, rendering historical trends—and the models that learned them—moot. Resilience was a requisite for survival and not many companies were equipped with the tools required to be agile.
When ML experiments drift off
Table of Contents
Machine learning models make predictions by finding patterns in data. But when that data becomes stale and no longer reflects the state of the world—a problem called “drift” occurs—so too do the models built on it. This can happen dramatically and at once, as it did with coronavirus, or more subtly: the customers you were targeting on Instagram move to TikTok; interest rates drop and the profile of a homebuyer becomes more millennial.
The impact on business is profound. To avoid this, you need to keep track of whether or not your models are stale. But knowing which of your models are in use and what they are doing is something many companies struggle with. Consider several features all drifting at the same time. This might seem like simple housekeeping compared to the hard math of building neural networks, but maybe that’s why it’s so often overlooked.
If training a model is like getting engaged, then using it effectively in production is like planning the wedding: a lot more complicated than you think it should be. From managing datasets to monitoring models to building processes that are shareable and repeatable throughout an organization, there are lots of moving parts to keep track of. The good news is that recently, many of these best practices have been codified into a new field: Machine Learning Operations or MLOps (the data-driven cousin of DevOps).
What’s more, because so much machine learning development has moved to the cloud, data science veterans like Google now offer opinionated tools that enable your own data science teams to follow those best practices without having to think about them.
MLOps best practices solve AI production hurdles
At Google I/O, we recently launched Vertex AI, a comprehensive managed ML platform that increases the rate of experimentation and accelerates time to business value for AI projects. With Vertex AI we are making AI more accessible and useful by:
- Shortening development cycles, and as a result, decreasing time to market
- Improving collaboration between teams across all levels of technical expertise
- Increasing reliability, performance, scalability, and security of ML systems
- Streamlining operational and governance processes
- Increasing return on investment of ML projects
For example, Vertex AI requires nearly 80% fewer lines of code to train a model versus competitive platforms, enabling data scientists and ML engineers across all levels of expertise the ability to implement MLOps to efficiently build and manage ML projects throughout the development lifecycle. This collaboration between technical and non-technical users alike empowers everyone to have a voice in the development process.
Global advertising agency, Essence is a Vertex AI customer and is using AI to help it stay on the cutting edge of creativity, innovation, and talent. Data is a constant influence in their decision-making. “Vertex AI gives our data scientists the ability to quickly create new models based on the change in environment while also letting our developers and data analysts maintain models in order to scale and innovate. The MLOps capabilities in Vertex AI mean we can stay ahead of our clients’ expectations.”-Mark Bulling, SVP, Product Innovation at Essence.
ModiFace, a part of L’Oréal, is a global market leader in augmented reality and artificial intelligence for the beauty industry. ModiFace creates new services for consumers to try beauty products such as hair color, makeup, and nail color, virtually, in real-time. ModiFace is using Vertex AI platform to train its AI models for all of its new services. For example, ModiFace’s skin diagnostic is trained on thousands of images from L’Oréal’s Research & Innovation, the company’s dedicated research arm. Bringing together L’Oréal’s scientific research combined with ModiFace’s AI algorithm, this service allows people to obtain a highly precise tailor-made skincare routine.
“We provide an immersive and personalized experience for people to purchase with confidence whether it’s a virtual try-on at web check out, or helping to understand what brand product is right for each individual,” said Jeff Houghton, chief operating officer at ModiFace, part of L’Oréal. “With more and more of our users looking for information at home, on their phone, or at any other touchpoint, Vertex AI allowed us to create technology that is incredibly close to actually trying the product in real life.”
Customers in the financial services industry are seeing similar business benefits to operationalizing their ML workloads—namely speed and scale. C6 Bank is using Vertex AI to quickly move from experimentation to production and Digits Financial needed an ML platform that would keep pace with its transaction volume as it scales. Deutsche Bank was able to use Google Cloud’s Vertex AI during a recent hackathon that aimed to use AI to improve the accessibility of its services to clients.
“Overall, we were impressed by the speed and ease of building custom models. We were able to build some of the services, and see the initial results within a day of experimenting with the product. Going forward, we see value in having a unified workflow with all the tools and services needed by our developers and data scientists.”-Mike Kellet, CTO Small Cap Bank, Deutsche Bank.
MLOps drives business growth
Today, we expect the software we build our business on to be scalable, reliable, and efficient. And if we want to reap the benefits of AI, we’ll need the same to be true of the models that increasingly drive business decisions. For years, we’ve optimized the way we build, run, and maintain software through DevOps, and now it’s time to do the same for machine learning. It’s uncharted territory for many, but at Google, we’ve learned how to make AI work at scale through years of trial and error—with MLOps—and it will ensure your business derives the most value from your investments in machine learning.
Related: Join us for the Google Cloud Applied ML Summit and learn how to apply groundbreaking machine learning technology to your business. Register here.