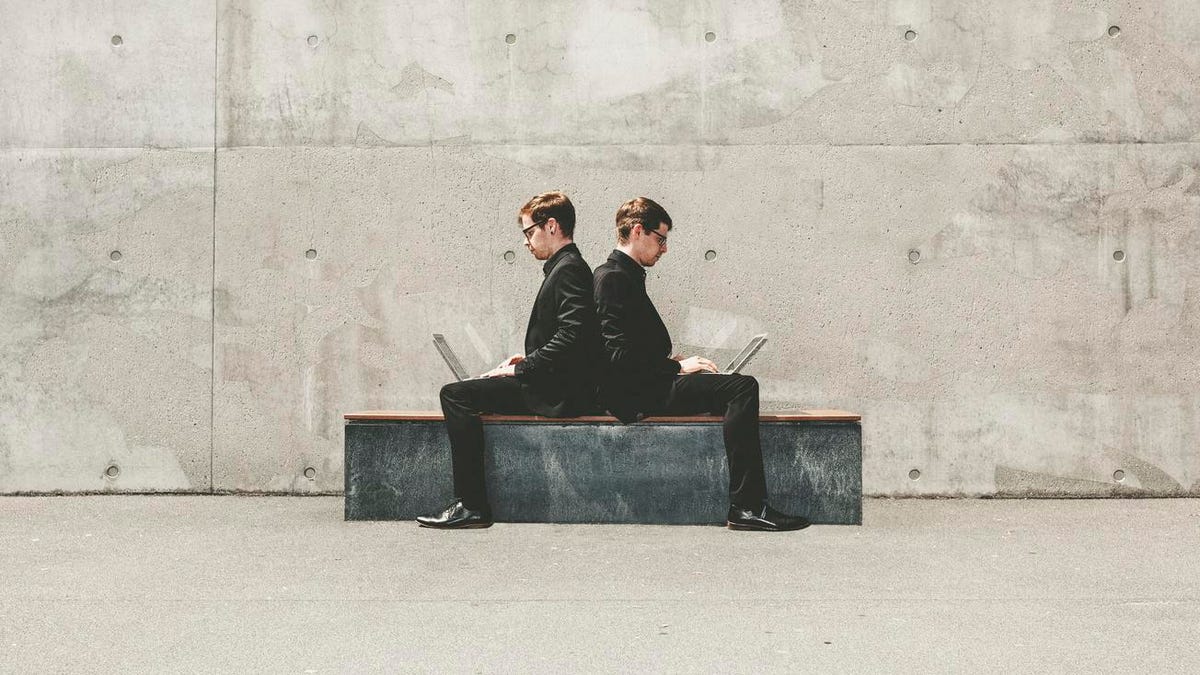
The precision that modern, cloud-enabled digital twins can offer is often a significant upgrade over older analytics methods.
A digital twin is a 1:1 software representation of a real-world object or system that is used to make predictions using simulated variables.
getty
Over the last few years, the “simulation hypothesis” has become an increasingly popular trope in online culture, promoting the idea that all of human existence—everything we experience—is in fact a simulation created by some superior intelligence for research purposes unknown.
I’m not here to tell you if this theory is correct—and anyone who claims such authority is of course lying. But less existentially and speculatively, a similar technology is actually making waves in the real world: digital twins.
A digital twin is a software representation of some real-world object or system. Often used for predictions or simulations, digital twins are constructed around 1:1 matches between data that impacts the object or system in reality and simulated variables that impact the simulated object.
For example, and as I have written before, autonomous vehicles can be difficult to train because it can be dangerous to collect certain kinds of data, such as self-driving car behavior to avoid road accidents. But by creating a digital twin of the vehicle and the environment in which it is driving, automakers can simulate how a car might interact with these variables—and then use these insights to refine their real-world product.
Driving simulations are only the tip of the iceberg when it comes to what digital twins are capable of. In the not-too-distant future, digital twins will play important roles in many industries, from banking to manufacturing, and even revolutionize complicated activities via mixed reality applications.
How digital twins have evolved
Table of Contents
Basic digital twins encompass any kind of real-world data point that is digitally replicated for the purposes of keeping track or making predictions, and some of the most sophisticated digital twins include detailed 3D models which are like the computer-generated special effects we’ve seen for decades in movies.
When a dinosaur convincingly stomps across the screen in a film, multiple simulations are involved: an underlying musculature system to make muscles expand and tense; texture and environmental simulations to govern how the skin moves, stretches, and reacts to light; and so on. But we’ve all seen movie effects that have a less convincing relationship to physics and reality, such as crashed cars that flip in impossible directions or rubbery digital stunt doubles that move in ways no human could. Some of this is artistic license but some of it is also indicative of poor simulations.
Several recent advances differentiate the digital twins used in the industry today from those in Hollywood’s more dubious special effects. Simulating interactions among many variables is computationally intensive, and prohibitively expensive if millions or billions of variables are involved. Cloud services help lower this barrier with affordable elastic computing and storage, which removes many of the marginal costs involved in creating simulations with more variables. Similarly, sophisticated cloud services that make machine learning accessible can support more sophisticated twins that simulate more complicated interactions.
At the same time, there’s been an explosion in data sources, including all kinds of sensors that continually generate real-time measurements. From sensors that track how components in machines are performing to those in wearable devices that measure heart rates or oxygen levels, both the number of variables we can measure and the depth in which we can measure them have grown exponentially—and ultimately, more data from more variables leads to smarter, more predictive and precise simulations.
Imagine designing an aircraft engine a decade ago, reliant on abstract math or limited simulations. Now imagine designing this engine today, with the ability to construct a digital twin of the engine and measure virtually infinite combinations of real-world telemetry data. The difference is astronomical!
The next generation of digital twins
The precision that modern, cloud-enabled digital twins can offer is often a significant upgrade over older analytics methods.
In the past, a manufacturer might know that on average, machines need service after nine months—so on a regular schedule, at meaningful cost, they shut down all the machines and service them.
Today, that manufacturer’s machines might be affixed with sensors and microphones that capture every vibration or mechanical groan. This data can be added to a digital representation of the machine—the digital twin. With smart modeling enabled by the cloud and constantly-refreshed data to refine those models, the manufacturer can simulate which machines are most likely to need service, and then efficiently shut down only those units, resulting in significant cost savings.
Or imagine what’s possible as wearable technologies such as smart glasses become more refined and ubiquitous. A utility worker wearing such glasses while inspecting equipment could see a digital overlay of the equipment they’re working on, able to interact with a replica and pull up relevant information even as they’re interacting with the physical machines.
Related: New research reveals what’s needed for AI acceleration in manufacturing
And the examples go on. Beyond maintenance, digital twins are already impacting manufacturing via computer-aided design (CAD), with engineering done first in digital space before physical prototyping occurs. Smart infrastructure is changing the way city governments understand and manage energy usage, traffic flows, pollution, and other factors, with insight into everything from how often each traffic meter is used to whether a construction project will delay public transit.
Banks’ ability to detect fraud is growing more precise, helping them to keep customer accounts safe. This can be done leveraging individualized models for user behavior, or in other words, digital twins of these bank customers. When insights go from general to granular, the variety and depth of possible applications expands by orders of magnitude.
Building a safe, simulated future
The prerequisites for the widespread use of digital twins are largely in place. As with most use cases involving analysis of data at massive scale, transparency, and respect for user privacy will be paramount to the adoption of many emerging use cases. A piston in a machine doesn’t care how many times it’s measured or by whom, but most people are going to have more exacting standards about their personal data.
As new mechanisms come online, we will gain new ways of understanding and interacting with our world by representing it in digital space. Whether or not we live in a simulation, bring on the digital twins!
A modern data warehouse can help you stay ahead of changing business needs. Download our future of data warehousing ebook to determine the best data warehouse for your needs.