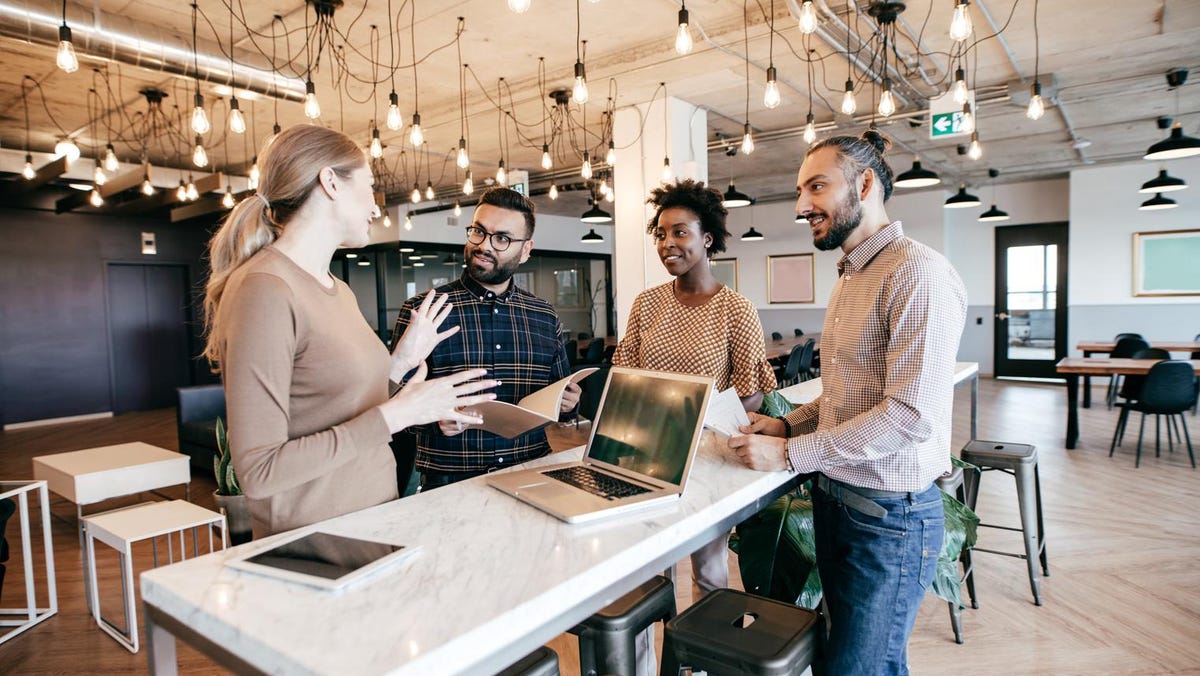
Travel technology company Sabre shares what they look for when hiring, along with tips on how ML professionals can set themselves up for career advancement.
It’s essential that data scientists and machine learning professionals are able to tell a good story when it comes to delivering complex technological solutions. This enables customers to understand how ML and AI can be valuable to their own processes.
getty
When it comes to the field of data science, artificial intelligence (AI), and machine learning (ML), skills are in greater demand than ever before. But as the industry evolves, ML practitioners must stay abreast of critical new skills in order to keep pace with innovation.
To learn more about what it takes to stay relevant in ML, we recently asked leaders of established ML teams in the world what they look for when hiring, the best ways to keep ML skills sharp, and how professionals can set themselves up for success, promotion, and career advancement.
In this particular Q&A, I had the chance to speak with Caroline Wester, Director of Engineering at travel technology company Sabre.
Sabre provides AI-driven solutions to airlines, hoteliers, agencies, and other travel partners around the world. The company’s technology has been on the cutting edge for decades, and Caroline’s team is working on solutions that are revolutionizing the industry, including voice recognition, advanced data analytics, and artificial intelligence.
Here’s what she had to say.
Insights from Sabre: What it takes to stay relevant in machine learning
Table of Contents
- 1 Insights from Sabre: What it takes to stay relevant in machine learning
- 1.1 1. What are the specific characteristics you look for when hiring a data scientist or another AI, deep learning, or machine learning professional?
- 1.2 2. You’ve mentioned several new skills like storytelling and explainable AI. How can a data scientist keep pace with technology changes and the overall pace of the industry at large?
- 1.3 3. As a follow-up topic, how much should people be thinking of learning and improving skills as “after work” versus “during work”? In other words, is learning something you do in your free time, or is it more of a structured concept that happens in an organization?
- 1.4 4. How are you thinking about developing talent in the future?
- 1.5 5. What’s one sentence of advice you would give up-and-coming talent in this field?
1. What are the specific characteristics you look for when hiring a data scientist or another AI, deep learning, or machine learning professional?
We’re looking for a couple of different things at Sabre. Certainly, having somebody that’s schooled in creating algorithms and understanding the deep technical knowledge is important.
But also, we need somebody that has the passion to learn the business and really knows how to apply the algorithms in a way that makes a difference to the business value that we’re trying to achieve.
It’s essential that people are able to tell a good story when it comes to delivering complex technological solutions, so our customers can understand how ML and AI can be valuable to their processes.
In addition, we’re also looking more at explainable AI. Models have to be explainable and produce certain insights that can be interpretable by the other functions within the organization. This allows us to justify why we take certain marketing or risk-credited decisions.
Related: Why you need to explain machine learning models
2. You’ve mentioned several new skills like storytelling and explainable AI. How can a data scientist keep pace with technology changes and the overall pace of the industry at large?
One of the things that I think is really important as somebody progresses through their career is to keep learning different things—not only learning from books but really diving into practical information and being able to bring that back to their day job.
Learning platforms and tools like Pluralsight and Coursera are great for being able to learn, but I think it’s really important for an engineer of any kind to dig deep into understanding a topic and then use it in their practical day-to-day work. This way, they can show that not only do they understand it from a book standpoint, but also from a practical standpoint. I encourage my engineers to do this. For anyone out there in the field, there’s a lot of opportunity to share information with your peers and learn from your peers—to not only understand how other people have learned this technology, but how they are applying it in their business.
Related: Google Cloud is partnering with Coursera to launch an ML Academy to sharpen your ML skills and learn about the latest ML technologies. Get started.
3. As a follow-up topic, how much should people be thinking of learning and improving skills as “after work” versus “during work”? In other words, is learning something you do in your free time, or is it more of a structured concept that happens in an organization?
One of the things I think is helpful is that we do a hackathon every quarter. It lasts about a week, and the advantage is that everyone is doing it at the same time. In my experience, formally designating time for everyone to participate as a group during work hours is much more productive. That way it doesn’t feel like an obligation on top of one’s day job. It enables teams to really focus on learning without being pulled into other things.
Understanding what the cloud can offer is incredibly critical and really what I look for in employees that I’m hiring. Moving forward, it’s not just the algorithms or the data and math skills—it’s really being able to apply that in the cloud.
When we do a week-long hackathon every quarter, we find that everyone participates. This really amplifies the collaboration, creativity, and focus around a few specific projects. Teammates receive concentrated support on exciting new ideas, all while furthering their technical skills and learning from what others have done.
At the same time, I think sometimes you need space from day-to-day work. For that reason, I also like to encourage people to explore outside the constraints of the organization.
4. How are you thinking about developing talent in the future?
My feeling is that the cloud is going to be so important and will continue to be important. Having those cloud skills is just going to be critical to data scientists, software developers, and really anyone in the business. That means not only understanding how to do machine learning on the cloud, but also how you deploy it. For example: Do you use TensorFlow? What tools are out there and what are the best practices to follow? How do you make sure that your models can be updated and how do you ensure your algorithms are learning as you go through the technology?
Cloud has really empowered our team to be more creative and helped to remove the challenges and technical complexity that holds some people back. For example, we can see our colleagues from other parts of the business are now interested in machine learning and data science. Cloud allows us to really put them at a level where they are harnessing AI and ML to contribute to the organization in new and meaningful ways.
Understanding what the cloud can offer is incredibly critical and really what I look for in employees that I’m hiring. Moving forward, it’s not just the algorithms or the data and math skills—it’s really being able to apply that in the cloud.
5. What’s one sentence of advice you would give up-and-coming talent in this field?
Get a mentor—find someone that is doing the job you want to do and stay close. Learn from them and build your business value through everything you do.
Stream this next: Learn how to apply groundbreaking machine learning technology in your projects. Register for our Applied ML Summit to watch sessions on demand.