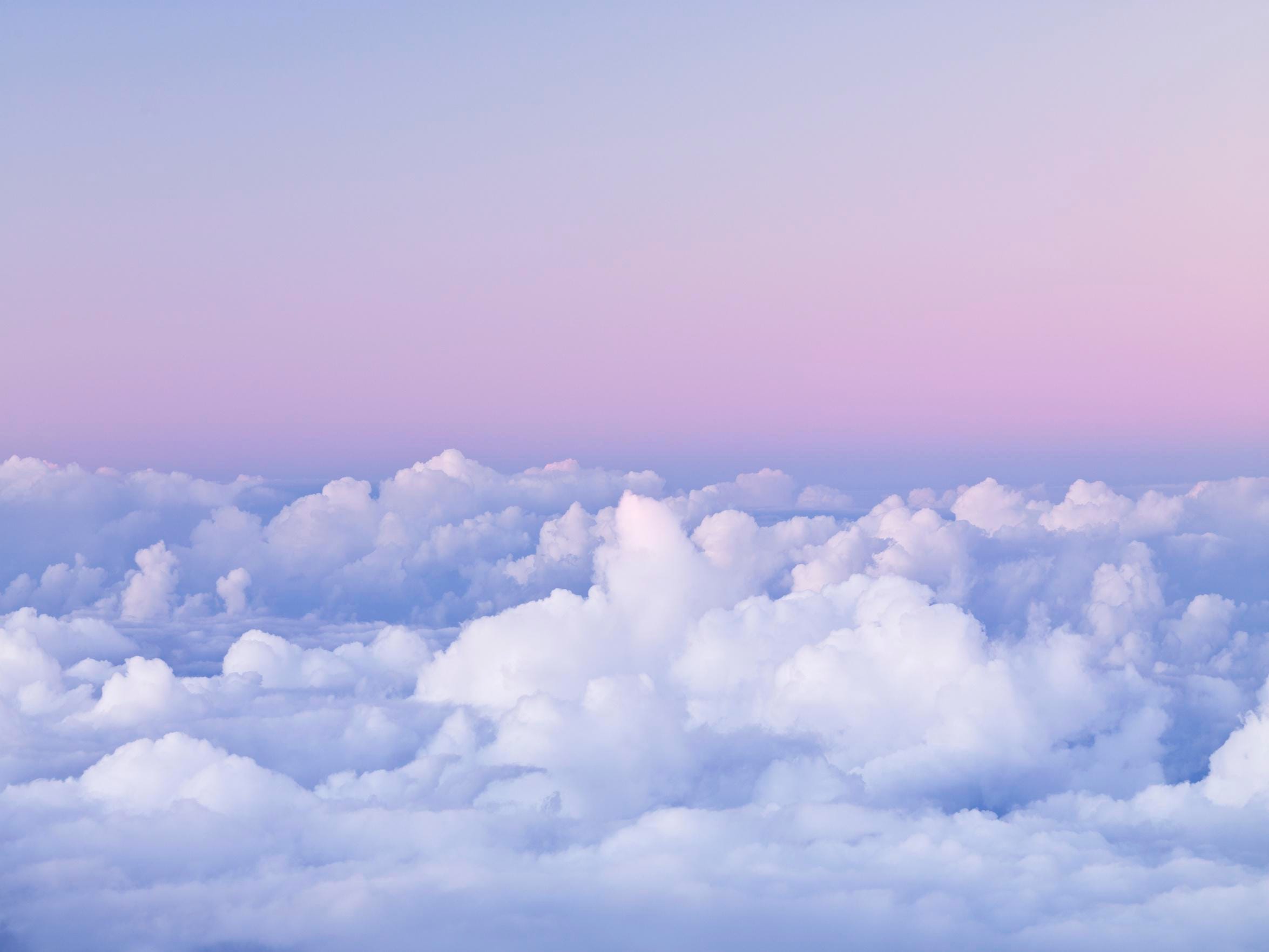
With data distributed across environments, cross-cloud analytics is more important than ever.
Most enterprises have multicloud architectures today. Their analytics tools should reflect that reality.
getty
As enterprises digitally transform their organization and workflows, the breadth of their software applications, data, and digital infrastructure expands. Workflows, teams and data often end up distributed across multiple environments – some stay in on-premise data centers, and many end up operating out of multiple cloud service providers.
A multicloud architecture is the present and future for most enterprises. Research shows that over 90% of large organizations already deploy multicloud architectures, and their data is distributed across several cloud providers. This reality presents several challenges to data teams within these organizations:
- Data silos – With applications hosted in multiple clouds and proliferation of data, data ends up in silos across data centers and cloud services, making it difficult for analysts to view, access and analyze organization-wide data through a single cohesive interface.
- Complexity of analytical tools – Each cloud platform vendor provides a unique set of analytical tools. The lack of uniformity between these tools makes it difficult for data teams to analyze efficiently.
- Data duplication – Moving large data sets across clouds to combine and create insights often leads to the duplication of data. Large scale data transfers are also expensive and risky.
While breaking down data silos is a top priority for many, it’s not often easy or straightforward to work with distributed data due to cost, security, governance and policy-related challenges.
So, how can organizations harness the power of data in a multicloud world? Data silos have been a pressing concern for enterprise CIOs for over a decade, yet they continue to increase in volume and complexity. To take advantage of the value of data spread across multicloud architectures, enterprises must fundamentally change the way they seek out that value.
The multicloud era requires a new approach. Rather than trying to eliminate silos by building massive data pipelines in which all data is copied from one place to the next and then sent back to where it came from, organizations must accept that silos will always exist. Data teams must stop trying to ETL their way into insights, and start getting more sophisticated in the way they query data. In other words, instead of ‘consolidating’ data silos, businesses should ‘cooperate’ data silos. They need to explore ways to look at their disparate data silos holistically so they are able to see all their data in a unified way through a single interface.
See you in the cloud.
Google Cloud
If organizations truly want to simplify their data landscape, they need to enable users to easily view data across multiple cloud environments and regions, to analyze data where it resides, to move just enough data for advanced analytics. This approach is called cross-cloud analytics. It’s a seamless way to view, combine, and analyze data across clouds through a single pane of glass to minimize problems associated with data silos.
Over the next few years 90% of data analytics workloads are expected to shift to cloud. Organizations that make that shift with a single pane of glass and cross-cloud analytics approach in mind will create an advantageous competitive position for themselves, rather than having to retrofit a strategy after their migration is complete. The following tenets provide a basis for an effective cross-cloud analytics strategy:
- Deploy multicloud-native tools that unify the management of core infrastructure and applications across on-premises, edge, and public clouds at scale.
- Create an intelligent data fabric that allows analysts to discover and analyze all data assets across organizations regardless of where or how it is stored. In the same way that operating systems made computers more approachable for all users, a data fabric makes enterprise data silos easily visible and more approachable for all analysts.
- Enforce consistent security policies. As more data assets are created, providing the correct level of access can be challenging. Security teams need to balance the risk of empowering their analysts while still controlling access to sensitive data. Security models that are centrally managed but customized between user domains provides the best of both worlds.
- Provide a single pane of glass to view and analyze data: Provide customers a single interface to view and analyze data irrespective of the cloud environment and region it resides in. An infrastructure that runs across multiple clouds not only reduces operational overhead but also reduces the inherent security risk of moving and duplicating data from one place to another.
- Future proof your data investments. By enabling data services that analyze existing data assets with minimal migration, create flexibility for being able to always choose the “best in breed” solution.
Benefits of cross-cloud analytics include:
- A consistent experience for data teams with the ability to access and query data across clouds through a single user interface
- Reduce the need for data engineering before analyzing data
- Lower operational overhead and risks by deploying an application that runs across multiple clouds which leverages the same, consistent security controls
- Faster access to insights by significantly reducing the time for data processing and analysis
- Consistent and predictable budgeting across multiple cloud footprints
- Enable long term agility and maximize the benefits every cloud investment
As data complexity increases, so do the costs and risks of management across multiple cloud environments. But so too does the value of being able to glean insights across these disparate sources. In the next 2-5 years we will see an explosion of use cases for cross-cloud analytics, from logistics and supply chains, to retail, manufacturing, travel, media, entertainment, and more. Enterprises that embrace this shift and build for cross-cloud analytics today will be the ones that best capitalize on these opportunities in the future.
Data Cloud Summit 2020
Google Cloud