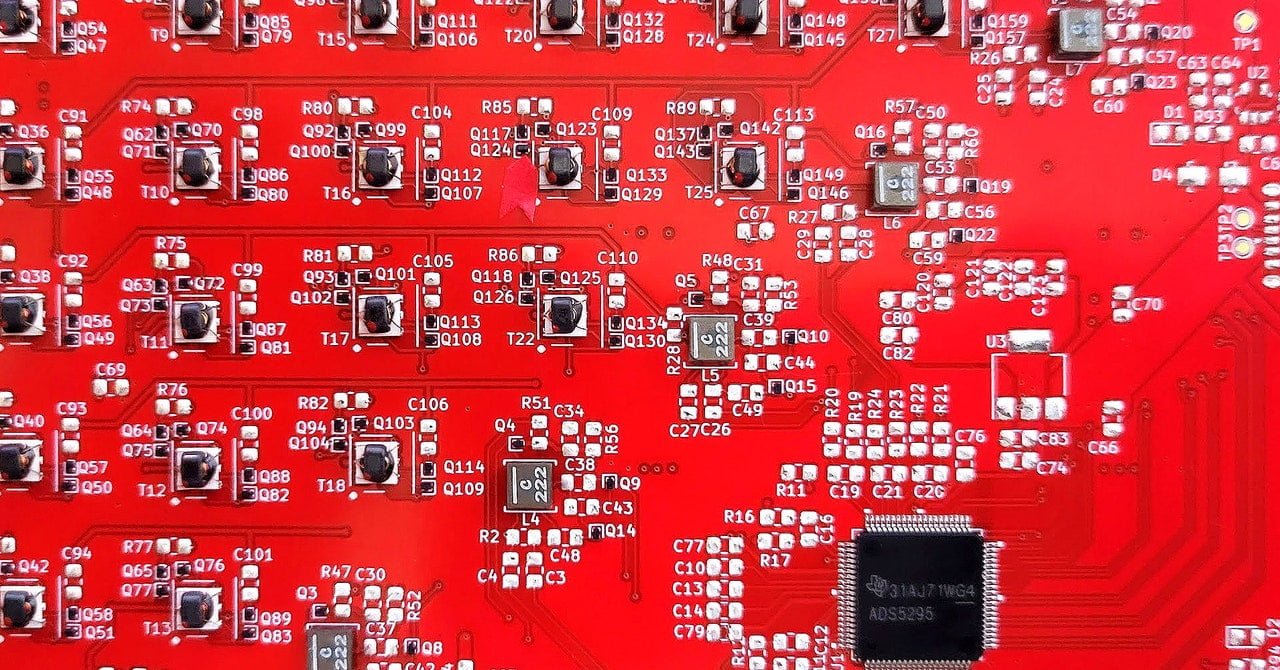
The cost of making further progress in artificial intelligence is becoming as startling as a hallucination by ChatGPT. Demand for the graphics chips known as GPUs needed for large-scale AI training has driven prices of the crucial components through the roof. OpenAI has said that training the algorithm that now powers ChatGPT cost the firm over $100 million. The race to compete in AI also means that data centers are now consuming worrying amounts of energy.
The AI gold rush has a few startups hatching bold plans to create new computational shovels to sell. Nvidia’s GPUs are by far the most popular hardware for AI development, but these upstarts argue it’s time for a radical rethink of how computer chips are designed.
Normal Computing, a startup founded by veterans of Google Brain and Alphabet’s moonshot lab X, has developed a simple prototype that is a first step toward rebooting computing from first principles.
A conventional silicon chip runs computations by handling binary bits—that’s 0s and 1s—representing information. Normal Computing’s stochastic processing unit, or SPU, exploits the thermodynamic properties of electrical oscillators to perform calculations using random fluctuations that occur inside the circuits. That can generate random samples useful for computations or to solve linear algebra calculations, which are ubiquitous in science, engineering, and machine learning.
Faris Sbahi, the CEO of Normal Computing, explains that the hardware is both highly efficient and well suited to handling statistical calculations. This could someday make it useful for building AI algorithms that can handle uncertainty, perhaps addressing the tendency of large language models to “hallucinate” outputs when unsure.
Sbahi says the recent success of generative AI is impressive, but far from the technology’s final form. “It’s kind of clear that there’s something better out there in terms of software architectures and also hardware,” Sbahi says. He and his cofounders previously worked on quantum computing and AI at Alphabet. A lack of progress in harnessing quantum computers for machine learning spurred them to think about other ways of exploiting physics to power the computations required for AI.
Another team of ex-quantum researchers at Alphabet left to found Extropic, a company still in stealth that seems to have an even more ambitious plan for using thermodynamic computing for AI. “We’re trying to do all of neural computing tightly integrated in an analog thermodynamic chip,” says Guillaume Verdon, founder and CEO of Extropic. “We are taking our learnings from quantum computing software and hardware and bringing it to the full-stack thermodynamic paradigm.” (Verdon was recently revealed as the person behind the popular meme account on X Beff Jezos, associated with the so-called effective accelerationism movement that promotes the idea of a progress toward a “technocapital singularity”.)
The idea that a broader rethink of computing is needed may be gaining momentum as the industry runs into the difficulty of maintaining Moore’s law, the long-standing prediction that the density of components on chips continues shrinking. “Even if Moore’s law wasn’t slowing down, you still have a massive problem, because the model sizes that OpenAI and others have been releasing are growing way faster than chip capacity,” says Peter McMahon, a professor at Cornell University who works on novel ways of computing. In other words, we might well need to exploit new ways of computing to keep the AI hype train on track.
That Normal, Extropic, and others trying to rethink the fundamentals of the computer chip are finding investors suggests that GPUs may be getting some competition relatively soon. Vaire Computing, a startup based in the UK, is developing silicon chips that work in a fundamentally different way to conventional ones, performing calculations without destroying information in the process. This approach, known as “reversible computing,” was devised decades ago and promises to make computing far more efficient but never took off. Vaire’s cofounder and CEO, Rodolfo Rosini, believes that physical limits of etching ever-smaller components into silicon means that GPUs and other conventional chips are running out of time. “We have one order of magnitude left” in chip manufacturing, Rosini says. “We could make components smaller, but the number one enemy is removing heat from the system fast enough.”
Convincing a huge industry to abandon a technology it has grown on top of for more than 50 years won’t be easy. But for the company that provides the next hardware platform, the payoff would be huge. “Every so often something comes along which will be transformative for the whole of humanity, like jet engines, transistor microchips, or quantum computers,” says Andrew Scott of 7percent Ventures, which is backing Vaire. The investors betting on Extropic’s and Normal’s own reimaginings of computing have similar hopes for their own contenders.
Even more exotic ideas—like moving away from using electricity inside computer hardware—are also gaining traction. McMahon’s own lab is looking at how to compute information using light to save energy. At a conference that he helped organize recently in Aspen, Colorado, a group of researchers from Holland showed off an idea for a mechanical cochlear implant that harnesses sound waves to power its computations.
It’s easy to dismiss chatbots, but the frenzy triggered by ChatGPT could be on track to incentivize revolutions in more than just AI software.
The Potential for a GPU Revolution Driven by ChatGPT’s Energy Consumption
Artificial intelligence (AI) has been rapidly advancing in recent years, with breakthroughs in natural language processing (NLP) leading to the development of powerful language models like OpenAI’s ChatGPT. These models have the ability to generate human-like text, making them useful for a wide range of applications such as chatbots, content creation, and virtual assistants. However, one major challenge in deploying such models at scale is their high energy consumption, primarily driven by the need for powerful graphics processing units (GPUs).
GPUs are specialized hardware that excel at performing parallel computations, making them ideal for training and running AI models. However, they are notorious for their high power consumption. This poses a significant problem as AI models like ChatGPT require extensive computational resources, resulting in substantial energy usage and associated environmental impacts.
The energy consumption of AI models has gained attention due to concerns about climate change and the need for sustainable technologies. OpenAI’s ChatGPT, for instance, has an estimated carbon footprint of roughly 626,000 pounds of CO2 emissions, equivalent to the lifetime emissions of nearly five average American cars. This highlights the urgent need to develop more energy-efficient alternatives to power AI models.
The potential for a GPU revolution lies in finding innovative ways to reduce energy consumption without compromising performance. Researchers and engineers are actively exploring various techniques to achieve this goal. One approach is to optimize the architecture of GPUs themselves. By designing more efficient GPUs that can perform AI computations with lower power requirements, it would be possible to significantly reduce the energy consumption of AI models.
Another avenue for reducing energy consumption is through algorithmic improvements. Researchers are constantly working on developing more efficient algorithms that can achieve similar or better performance with fewer computational resources. This would not only reduce energy consumption but also make AI models more accessible and affordable for a wider range of applications.
Additionally, advancements in hardware acceleration technologies hold promise for energy-efficient AI. Field-programmable gate arrays (FPGAs) and application-specific integrated circuits (ASICs) are being explored as alternatives to GPUs. These specialized chips can be designed specifically for AI workloads, resulting in improved performance and energy efficiency.
Furthermore, distributed computing and cloud-based solutions can contribute to reducing energy consumption. By distributing the computational load across multiple machines or data centers, it is possible to optimize resource utilization and minimize energy waste. Cloud providers are also investing in renewable energy sources to power their data centers, further reducing the carbon footprint of AI models.
The potential benefits of a GPU revolution driven by reduced energy consumption are immense. It would not only address environmental concerns but also enable wider adoption of AI technologies. Energy-efficient AI models would be more accessible to organizations with limited computational resources, fostering innovation and driving economic growth. Moreover, reduced energy consumption would lower operational costs, making AI more economically viable for businesses.
However, it is important to note that achieving a GPU revolution driven by energy efficiency is a complex task that requires collaboration between researchers, engineers, and policymakers. It involves striking a balance between performance, energy consumption, and cost-effectiveness. Additionally, efforts should be made to ensure that the transition to energy-efficient AI does not compromise the quality and capabilities of AI models.
In conclusion, the potential for a GPU revolution driven by reduced energy consumption holds great promise for the future of AI. By optimizing GPU architecture, developing more efficient algorithms, exploring alternative hardware acceleration technologies, and leveraging distributed computing and cloud-based solutions, it is possible to significantly reduce the energy footprint of AI models like ChatGPT. This would not only address environmental concerns but also unlock new opportunities for innovation and economic growth.