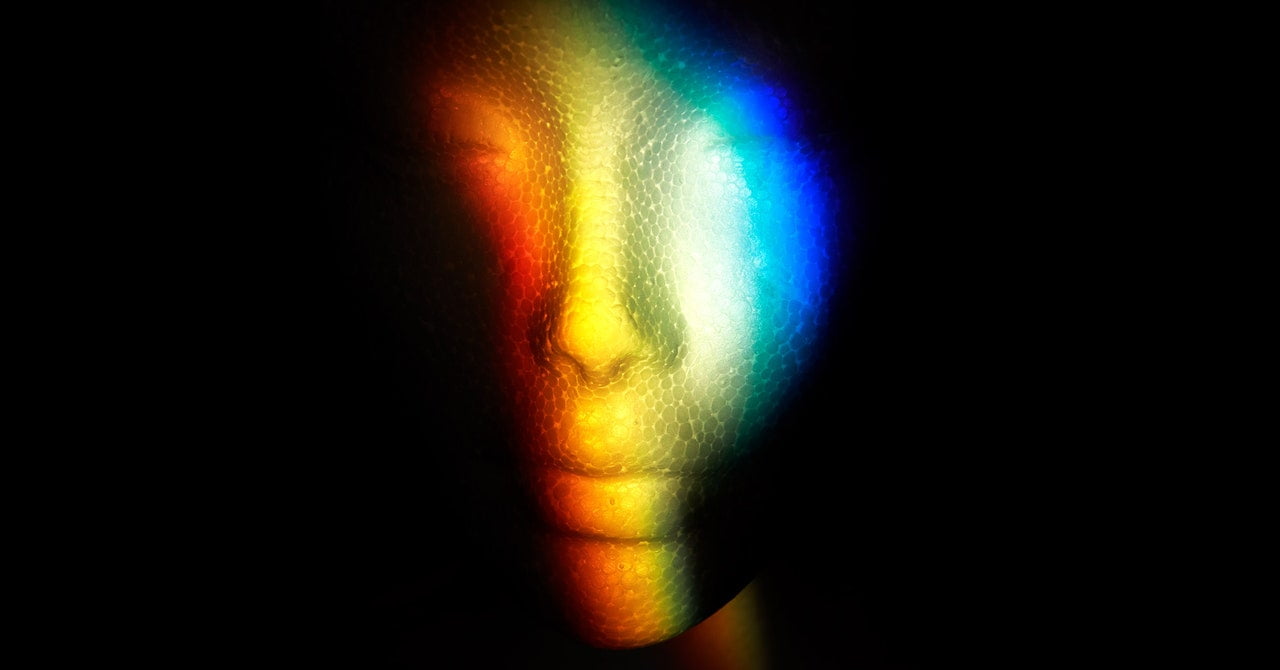
When OpenAI published details of the stunningly capable AI language model GPT-4, which powers ChatGPT, in March, its researchers filled 100 pages. They also left out a few important details—like anything substantial about how it was actually built or how it works.
That was no accidental oversight, of course. OpenAI and other big companies are keen to keep the workings of their most prized algorithms shrouded in mystery, in part out of fear the technology might be misused but also from worries about giving competitors a leg up.
A study released by researchers at Stanford University this week shows just how deep—and potentially dangerous—the secrecy is around GPT-4 and other cutting-edge AI systems. Some AI researchers I’ve spoken to say that we are in the midst of a fundamental shift in the way AI is pursued. They fear it’s one that makes the field less likely to produce scientific advances, provides less accountability, and reduces reliability and safety.
The Stanford team looked at 10 different AI systems, mostly large language models like those behind ChatGPT and other chatbots. These include widely used commercial models like GPT-4 from OpenAI, the similar PaLM 2 from Google, and Titan Text from Amazon. The report also surveyed models offered by startups, including Jurassic-2 from AI21 Labs, Claude 2 from Anthropic, Command from Cohere, and Inflection-1 from chatbot maker Inflection.
And they examined “open source” AI models that can be downloaded for free, rather than accessed exclusively in the cloud, including the image-generation model Stable Diffusion 2 and Llama 2, which was released by Meta in July this year. (As WIRED has previously covered, these models are often not quite as open as they might seem.)
The Stanford team scored the openness of these models on 13 different criteria, including how transparent the developer was about the data used to train the model—for example, by disclosing how it was collected and annotated and whether it includes copyrighted material. The study also looked for disclosures about the hardware used to train and run a model, the software frameworks employed, and a project’s energy consumption.
Across these metrics, the researchers found that no model achieved more than 54 percent on their transparency scale across all these criteria. Overall, Amazon’s Titan Text was judged the least transparent, while Meta’s Llama 2 was crowned the most open. But even an “open source” model like Llama 2 was found to be quite opaque, because Meta has not disclosed the data used for its training, how that data was collected and curated, or who did the work.
Nathan Strauss, a spokesperson for Amazon said the company is closely reviewing the index. “Titan Text is still in private preview, and it would be premature to gauge the transparency of a foundation model before it’s ready for general availability,” he says. Meta declined to comment on the Stanford report and OpenAI did not respond to a request for comment.
Rishi Bommasani, a PhD student at Stanford who worked on the study, says it reflects the fact that AI is becoming more opaque even as it becomes more influential. This contrasts greatly with the last big boom in AI, when openness helped feed big advances in capabilities including speech and image recognition. “In the late 2010s, companies were more transparent about their research and published a lot more,” Bommasani says. “This is the reason we had the success of deep learning.”
The Stanford report also suggests that models do not need to be so secret for competitive reasons. Kevin Klyman, a policy researcher at Stanford, says the fact that a range of leading models score relatively highly on different measures of transparency suggests that all of them could become more open without losing out to rivals.
As AI experts try to figure out where the recent flourishing of certain approaches to AI will go, some say secrecy risks making the field less of a scientific discipline than a profit-driven one.
“This is a pivotal time in the history of AI,” says Jesse Dodge, a research scientist at the Allen Institute for AI, or AI2. “The most influential players building generative AI systems today are increasingly closed, failing to share key details of their data and their processes.”
AI2 is trying to develop a much more transparent AI language model, called OLMo. It is being trained using a collection of data sourced from the web, academic publications, code, books, and encyclopedias. That data set, called Dolma, has been released under AI2’s ImpACT license. When OLMo is ready, AI2 plans to release the working AI system and also the code behind it too, allowing others to build upon the project.
Dodge says widening access to the data behind powerful AI models is especially important. Without direct access, it is generally impossible to know why or how a model can do what it does. “Advancing science requires reproducibility,” he says. “Without being provided open access to these crucial building blocks of model creation we will remain in a ‘closed’, stagnating, and proprietary situation.”
Given how widely AI models are being deployed—and how dangerous some experts warn they might be—a little more openness could go a long way.