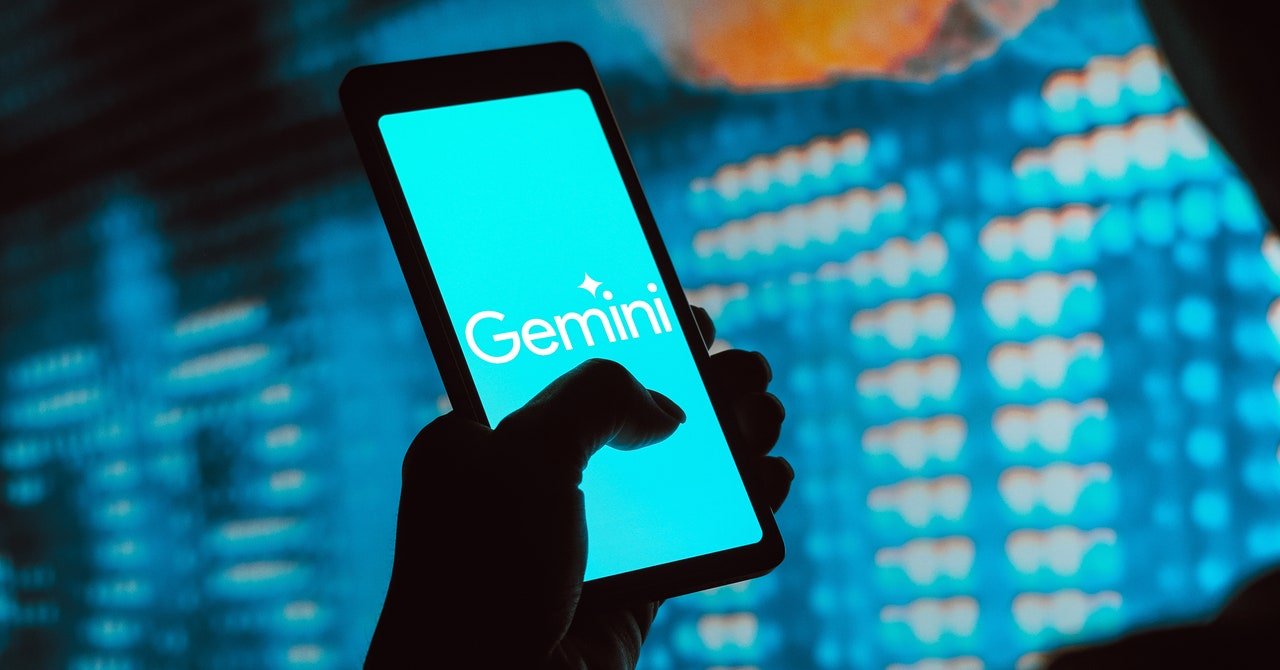
Google has admitted that its Gemini AI model “missed the mark” after a flurry of criticism about what many perceived as “anti-white bias.” Numerous users reported that the system was producing images of people of diverse ethnicities and genders even when it was historically inaccurate to do so. The company said Thursday it would “pause” the ability to generate images of people until it could roll out a fix.
When prompted to create an image of Vikings, Gemini showed exclusively Black people in traditional Viking garb. A “founding fathers” request returned Indigenous people in colonial outfits; another result depicted George Washington as Black. When asked to produce an image of a pope, the system showed only people of ethnicities other than white. In some cases, Gemini said it could not produce any image at all of historical figures like Abraham Lincoln, Julius Caesar, and Galileo.
Many right-wing commentators have jumped on the issue to suggest this is further evidence of an anti-white bias among Big Tech, with entrepreneur Mike Solana writing that “Google’s AI is an anti-white lunatic.”
But the situation mostly highlights that generative AI systems are just not very smart.
“I think it is just lousy software,” Gary Marcus, an emeritus professor of psychology and neural science at New York University and an AI entrepreneur, wrote on Wednesday on Substack.
Google launched its Gemini AI model two months ago as a rival to the dominant GPT model from OpenAI, which powers ChatGPT. Last week Google rolled out a major update to it with the limited release of Gemini Pro 1.5, which allowed users to handle vast amounts of audio, text, and video input.
Gemini also created images that were historically wrong, such as one depicting the Apollo 11 crew that featured a woman and a Black man.
On Wednesday, Google admitted its system was not working properly.
“We’re working to improve these kinds of depictions immediately,” Jack Krawczyk, a senior director of product management at Google’s Gemini Experiences, told WIRED in an emailed statement. “Gemini’s AI image generation does generate a wide range of people. And that’s generally a good thing because people around the world use it. But it’s missing the mark here.”
Krawczyk explained the situation further in a post on X: “We design our image generation capabilities to reflect our global user base, and we take representation and bias seriously. We will continue to do this for open ended prompts (images of a person walking a dog are universal!) Historical contexts have more nuance to them and we will further tune to accommodate that.”
He also responded to some critics directly by providing screenshots of his own interactions with Gemini which suggested the errors were not universal.
But the issues Gemini produced were quickly leveraged by anti-woke crusaders online, who claimed variously that Google was “racist” or “infected with the woke mind virus.”
Far-right internet troll Ian Miles Cheong blamed the entire situation on Krawczyk, whom he labeled a “woke, race-obsessed idiot” while referencing posts on X from years ago where Krawczyk acknowledged the existence of systemic racism and white privilege.
“We’ve now granted our demented lies superhuman intelligence,” Jordan Peterson wrote on his X account with a link to a story about the situation.
But the reality is that Gemini, or any similar generative AI system, does not possess “superhuman intelligence,” whatever that means. If anything, this situation demonstrates that the opposite is true.
As Marcus points out, Gemini could not differentiate between a historical request, such as asking to show the crew of Apollo 11, and a contemporary request, such as asking for images of current astronauts.
Historically, AI models including OpenAI’s Dall-E have been plagued with bias, showing non-white people when asked for images of prisoners, say, or exclusively white people when prompted to show CEOs. Gemini’s issues may not reflect model inflexibility, “but rather an overcompensation when it comes to the representation of diversity in Gemini,” says Sasha Luccioni, researcher at the AI startup Hugging Face. “Bias is really a spectrum, and it’s really hard to strike the right note while taking into account things like historical context.”
When combined with the limitations of AI models, that calibration can go especially awry. “Image generation models don’t actually have any notion of time,” says Luccioni, “so essentially any kind of diversification techniques that the creators of Gemini applied would be broadly applicable to any image generated by the model. I think that’s what we’re seeing here.”
As the nascent AI industry attempts to grapple with how to deal with bias, Luccioni says that finding the right balance in terms of representation and diversity will be difficult.
“I don’t think there’s a single right answer, and an ‘unbiased’ model doesn’t exist,” Luccioni said. “Different companies have taken different stances on this. It definitely looks funny, but it seems that Google has adopted a Bridgerton approach to image generation, and I think it’s kind of refreshing.”
Artificial intelligence (AI) has made significant strides in recent years, revolutionizing various industries and transforming the way we live and work. From self-driving cars to voice assistants, AI has become an integral part of our daily lives. However, the limitations of AI have also become apparent, as demonstrated by Google’s ‘woke’ image generator.
Google’s ‘woke’ image generator, known as Deep Dream, gained popularity in 2015 when it was released to the public. Deep Dream uses a neural network to analyze and modify images, creating surreal and dream-like visuals. Users can input an image, and the AI algorithm will enhance certain features, often resulting in bizarre and psychedelic patterns.
While Deep Dream was initially intended as a fun experiment, it inadvertently exposed some of the limitations of AI. One of the main limitations is the lack of contextual understanding. Deep Dream’s algorithm is trained on a large dataset of images, allowing it to recognize patterns and objects. However, it struggles to comprehend the meaning or context behind these images.
This limitation becomes evident when Deep Dream modifies images in unexpected ways. For example, if a user inputs an image of a dog, Deep Dream might enhance certain features to make it appear more dog-like. However, it can also introduce unrelated patterns or objects that have no relevance to the original image. This lack of contextual understanding highlights the challenge of teaching AI algorithms to comprehend images in a meaningful way.
Another limitation exposed by Deep Dream is the potential for bias and unintended consequences. AI algorithms are trained on vast amounts of data, which can include biases present in society. These biases can be inadvertently perpetuated by the AI algorithm, leading to unintended outcomes. In the case of Deep Dream, users noticed that certain patterns or objects were more likely to appear in modified images. This raised concerns about the underlying biases within the algorithm and the potential for reinforcing stereotypes or discriminatory imagery.
Furthermore, Deep Dream’s limitations shed light on the broader challenge of explainability in AI. While the algorithm can generate visually appealing images, it is often difficult to understand why it makes certain modifications. The neural network’s decision-making process is complex and opaque, making it challenging for users to comprehend or control the output. This lack of transparency can be problematic, especially in critical applications where accountability and understanding are crucial.
Despite these limitations, Google’s ‘woke’ image generator has sparked important discussions about the boundaries of AI. It serves as a reminder that AI algorithms are not infallible and require ongoing refinement and oversight. Researchers and developers must continue to address the limitations of AI to ensure its responsible and ethical use.
In conclusion, Google’s ‘woke’ image generator, Deep Dream, has exposed some of the limitations of AI. Its lack of contextual understanding, potential for bias, and challenges in explainability highlight the complexities involved in developing AI algorithms. While AI has made significant progress, it is essential to recognize and address these limitations to ensure its responsible and beneficial integration into society.