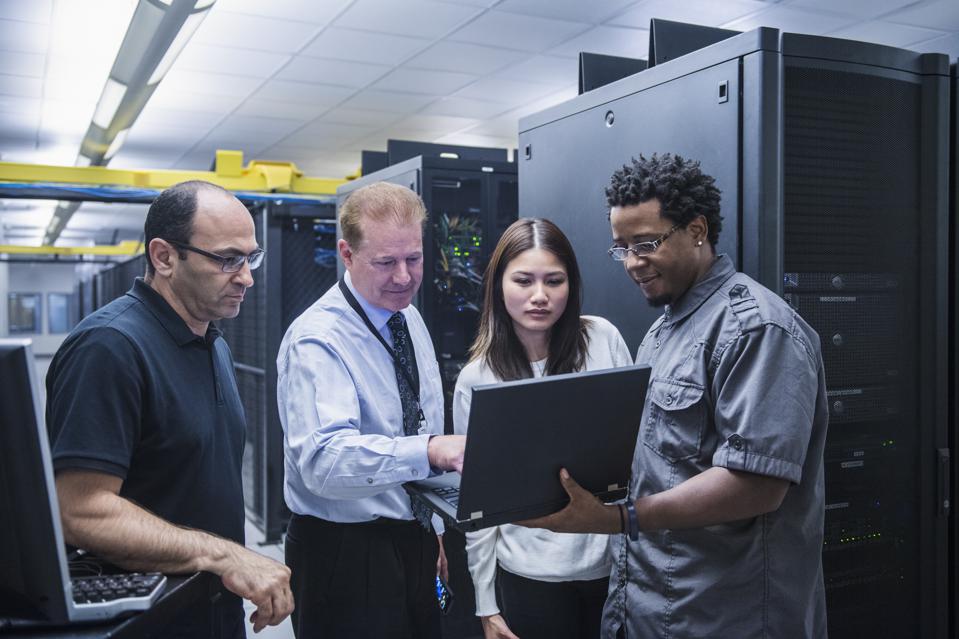
The transformational business results that come from being a data-driven company won’t materialize from a single gesture, but the results can drive the growth strategies that sustain and propel a business forward.
getty
Gambling your company’s future value on intuition isn’t a sustainable strategy—but getting what you need out of data can seem overwhelming. Here’s how you can tackle data technologies to meet business goals.
In recent years, as terms like “big data” and “real-time analytics” have invaded the enterprise lexicon, numerous analyses have reinforced that data-driven companies tend to outperform competitors. A recent Deloitte survey, for example, states that “Organizations that reported having the strongest cultural orientation to data-driven insights and decision-making are twice as likely to have reported exceeding business goals in the past 12 months.”
Despite this seemingly obvious fact, many companies still rely on intuition and questionable judgement—rather than data-driven decisioning—for much of their problem solving.
This can happen for a number of reasons: cultures that prioritize praising “winners” rather than those who make the best decisions; IT operations that don’t make data accessible or easy to interpret; the constant pressure to ship; the tendency to privilege personal experience over data; and so on.
Despite the challenges, building a data-driven company is not out of reach. Asking the right questions—and getting the right data to the right people—is the hard part. In this article, we’ll examine five practical steps a company can take to create a data-driven culture.
1. Get the data flowing.
Table of Contents
Newton’s first law of motion states that an object at rest will stay at rest unless acted on by an outside force. We should pattern a new first law of data after Newton’s observation, since corporate data in the enterprise works much the same way: Data at rest tends to stay at rest. Research has shown that data flow at the edge of a corporation’s network can predict financial performance—so it’s important to get data moving, and to create interactions around your data by getting it to the right people in the right contexts.
After all, if the value of shopping centers corresponds to the number of shoppers they attract, then digital interactions—the shoppers of the digital world—should drive increased value to the corporation in much the same way. The more digital footfall (i.e., the greater the usage of the application programming interfaces, or APIs, that connect data to applications and user experiences), the bigger the financial outcome should be. This is even further magnified by internal APIs, which predict sales more strongly than external APIs.
2. Make product decisions based on data.
This one seems obvious, right? One of the biggest challenges faced by large enterprises is that there is often no single source of data that can be leveraged. The data associated with customer interactions, for instance, must be aggregated from multiple elements—CRM systems, ads data, various application databases, log files, and more. Creating a single pane of glass—a focal point to aggregate and collect this data so that it is available to decision-makers—is a key step toward driving an objective decision-making process.
In the era of digital ecosystem competition, in which many services and customer experiences rely on technologies from multiple companies or platforms, single-pane access to actionable data is frequently married to not only data analytics products such as BigQuery, Google Cloud’s enterprise data warehouse, but also the API management platform. Since virtually all digital interactions pass through APIs, API management offers a single point at which all transactions and behaviors can be aggregated, analyzed, and leveraged, both through visualization tools and machine learning (ML) models.
Related: Does the key to business transformation start with your organization’s data culture? Get Google’s guide on building a data-driven culture.
3. Produce new data based on data.
The process—and promise—of delivering ML value to the enterprise is frequently challenged by the amount of data that needs to be used for training and then re-training. This is magnified by the emerging discipline of continuous delivery (CD) for ML modeling, in which models are constantly re-trained and released, producing even more data. It can be easy to focus simply on the volume and storage costs of data, but keep in mind the larger goals. Cost rationalization is important, but not at the expense of delivering new value to customers.
4. Put data in everyone’s hands.
Getting access to the right data isn’t just about APIs. Almost always, even if the appropriate APIs already exist, they are only available to developers who write software that consumes and leverages those APIs. A new set of tools, known as low-code or no-code platforms, democratizes access to data by targeting business users (sometimes referred to as “citizen developers”), empowering them to design, build, and deploy apps and business automations that harness the enterprise’s data.
This can help all parties that need to make data-driven decisions to do so, whether they are software developers, business analysts, operations managers, strategic negotiators, or virtually any interested party—and whether they need solutions for the office, for working from home, or for working in the field. Application design and development are no longer the exclusive purview of the developer, allowing anyone in the workforce to do more with data.
5. Lean in to strategic openness.
Many enterprises have historically shown reticence toward openness, keeping data within the corporate firewall and locking off access to digital assets. This is the equivalent of building corporate castles—replete with moats, drawbridges, and heavy castle walls—around the data: It might feel safe, but it makes it awfully hard to get the data moving. But what if the best security posture is actually to lean in to openness? Instead of locking down data, enterprises can build a security culture that identifies sensitive vs. non-sensitive information, provisions who is accessing what, and provides self-service access to data while still giving administrators the ability to restrict access to the right people.
This can help build a culture around developer (and citizen developer) enablement while still keeping data protected. In some organizations, data is siloed even between business units, impeding development and management of products that are sensitive to a 360-degree view of customer interactions. These impediments can lead to negative customer experiences, such as having multiple apps to log in to, multiple different usernames and passwords for a company’s apps, and even multiple different payment methods. For many enterprises, leveraging and contributing to an API catalogue is a great step toward changing the working model of a company from guarded, possessive, and nervous to collaborative, dynamic, and modern.
Becoming a data-driven company requires assessing an organization from multiple angles: infrastructure rationalization and developer engagement to strategic alignment, new KPIs oriented to encourage employees to do more with data, and potential network effects that may arise from expanded data efforts. The transformational business results that come from being a data-driven company won’t materialize from a single gesture, but the results can drive the growth strategies that sustain and propel a business forward.
Get a comprehensive analysis of 19 API management vendors. Access your complimentary copy of the 2020 Gartner Magic Quadrant for Full Life Cycle API Management.