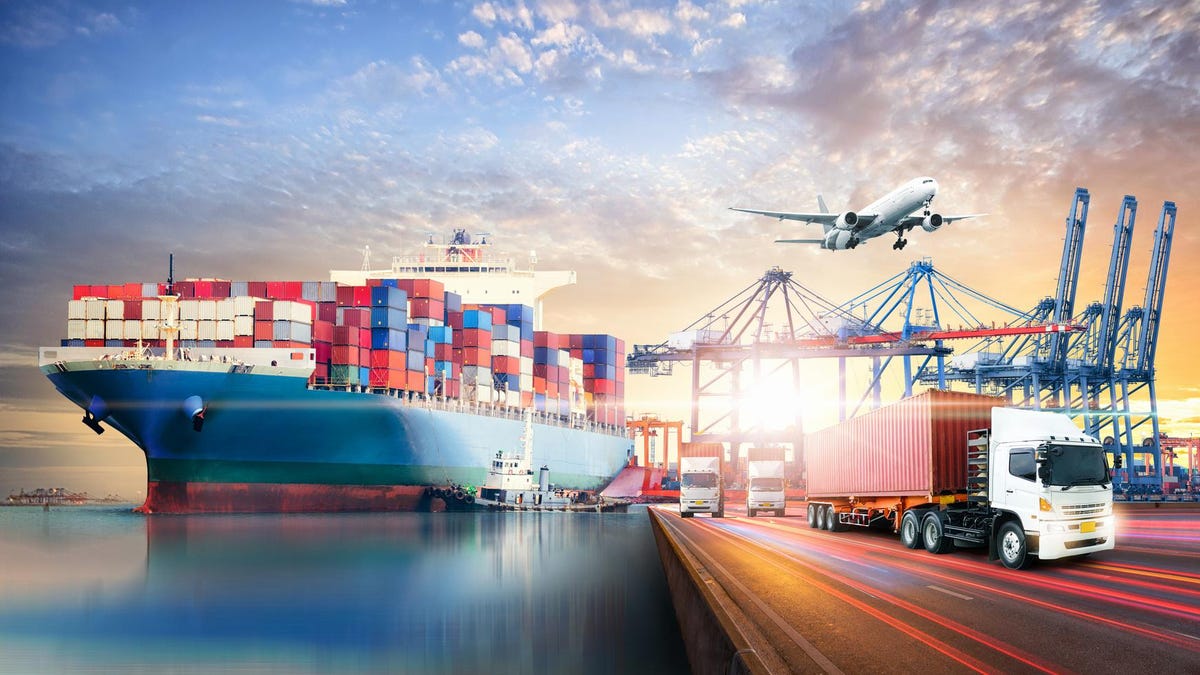
Ensuring supply chains are equipped for future disruptions and evolving challenges
Data management and insights driven by machine learning and artificial intelligence are critical to effective supply chain transformation and resiliency.
getty
By now, everyone has experienced multiple supply chain disruptions wrought by COVID-19, from the recent chip shortage to those months last year when toilet paper was near-impossible to find. The pandemic opened our eyes to the economic and human fallout when supply chains are stressed—but pandemics are not the only source of these disruptions.
Extreme weather events, changing geopolitical conditions, access to raw materials, and environmental impacts all posed significant risks even before the pandemic. To build resilient and sustainable supply chains going forward, we must holistically account for all of these factors.
In our How Data Is Driving Resilient Supply Chains whitepaper, we explore why data management and insights driven by machine learning and artificial intelligence (ML and AI) are critical to effective supply chain transformation. In too many supply chain models raw materials are eventually translated to waste, over the lifecycle of the products companies create. They also rely on fragile networks that can be crippled if one segment in the overall chain falters. A circular economy approach is required, with agile, flexible relationships across the supply chain; that decouples economic activity from the consumption of finite resources; and design practices that eliminate waste.
In this article, we’ll highlight some of the major themes of our new whitepaper, including what it looks like in practice to architect a resilient and sustainable supply chain.
Data is changing supply chains
Supply chains need data to provide visibility and intelligence across the network, enabling companies to optimize relationships, reduce environmental impact, and adapt to disruptions in real-time. This involves reimagining three core processes:
Supply chain design: Improving supply chain design involves transforming both product innovation— which predetermines most supply chain and sustainability costs—as well as network design. In the former case, businesses need more holistic insight into which materials, ingredients, and components are being used; which packaging material is required; where supplies are being sourced; what production processes and energy consumption are occurring; etc. Lacking this insight, businesses can make only point decisions. Network design, meanwhile, involves managing distribution networks as well as recycle and return processes—areas that, like those in product innovation, require comprehensive visibility into data from many sources, as well as AI and ML models to make the data actionable.
Supply chain monitoring: Most companies only have a connection to their direct suppliers, meaning they don’t know much about their suppliers’ suppliers. These limitations make it impossible to fully understand supply chain challenges, whether logistical or environmental, across the lifecycle of an enterprise’s output. Building an effective track-and-trace process therefore requires a network of companies sharing information, and it potentially may also include sources such as satellites for monitoring deforestation, water, agriculture, and mining. Additional technologies such as Blockchain, 5G, and sensors on pallets are important as well, as they help to provide secure, real-time connectivity to and visibility into chains of custody and network interactions. Collecting and managing all of this information requires a scalable data lake and the integration of many data sources.
Supply chain execution: This process involves risk prediction and disruption management. As with the previous two core processes, there’s a common thread: uniting data from many disparate sources so that decisions can be made holistically rather than in isolation, and applying ML to the data so that decisions can be smarter and faster. Currently, for example, companies tend to make short-term decisions based on cost impacts, without a broader view of sustainability variables.
Across these processes, a variety of data processing and management requirements become evident:
Build the collective data models: The first step is to model the business network, which includes multi-tier suppliers, logistics partners, manufacturing partners, equipment manufacturers, service providers, and of course customers. Likewise, all products, components, and inputs used in the network should be modeled.
Differentiate and monitor risk: Companies need to differentiate three major risk categories: natural (e.g., earthquakes, extreme weather); operational (e.g., variables affecting forecast accuracy, supply shortages, inventory positioning, and asset downtime); and financial (e.g., risk profiles of business partners).
Monitor sustainability: Most companies have started monitoring their greenhouse gas emissions, but to achieve a circular economy, companies must understand not only the emissions they cause but also their energy consumption (including how much comes from renewable sources) as well as their partners’ emissions and energy consumption. Likewise, social responsibility should become a prominent consideration, with enterprises monitoring deforestation, human rights conditions, the effects of mining, and other factors that intersect with their suppliers.
Leverage AI/ML algorithms: Risk management and sustainability processes currently focus on analytics and scoring mechanisms—the next step is to use AI and ML algorithms for product innovation, supply network design, risk prediction, and disruption management. By leveraging AI to dynamically optimize fleet routing, a global logistics provider was able to reduce their annual fuel consumption by 10 million gallons (approximately 38 million liters) and save over 400 million US dollars.
Architecting the digital supply chain of the future
Based on the requirements described above, we can identify four pillars for architecting a comprehensive digital supply chain platform for sustainable and resilient supply chains:
A digital twin of the supply chain: A digital representation of the physical supply chain, each company-specific twin helps enterprises to see and understand the relationships that make up their supply chain, detect opportunities for optimization, model and assess different changes, and make contingencies for natural disasters or other disruptions.
User access: Access to the digital twin’s information should be available to the appropriate supply chain personnel on all their devices, including functionality for users to receive alerts, analyze data, communicate with colleagues or business partners, and trigger simulations.
Supply chain simulation and optimization: Resilient and sustainable supply chain processes are huge data challenges, and AI and ML algorithms help companies significantly reduce risks and meet sustainability targets.
Supply chain partners: Few if any enterprises’ internal systems include all the data sets required to holistically visualize and manage supply chain risk and sustainability. Partner relationships, and the agility with which they can be managed, are essential.
Related: Attend the Digital Supply Chain Summit to learn how data drives resilient and sustainable supply chains, and get customized solutions for your business.
We believe this kind of architecture, with competencies based on these four pillars, will ensure that future supply chains are equipped for a variety of disruptions and rapidly-evolving challenges, whether it’s the short-term urgency of stockouts to the long-term mandate to conserve natural resources. To learn more, download our How Data Is Driving Resilient Supply Chains whitepaper.