Sentiments and emotions are hard to quantify, but, ironically, are the most crucial factors when a user makes a purchase decision. Users share their thoughts and opinions across various social platforms day-in-out. To make the most of it, brands need to monitor the chatter and derive useful insights that help understand user emotions, intent, and purpose. After all, every brand wants to know how their target users “feel” about them!
What is Social Sentiment Analysis?
Table of Contents
Marketers are obsessed with metrics, and why not. Metrics help you boost your business in the right direction. However, metrics cannot surpass your users’ sentiments and feelings.
Social sentiment analysis can help brands dig deep into how their target users “feel” about them and turn social insights into actionable data. When a brand tries to incorporate user perceptions into its marketing and branding strategy, you get a successful and loved brand.
Social sentiment analysis, or opinion mining, is a natural language processing technique that tells you if the data acquired is positive, negative, or neutral. This enables brands to create a better user experience. After all, it is the end-users experience that matters.
Sentiment Analysis + AI
AI tools are paving the way in every domain we can think of. Whether driving business goals, writing content, or generating customized audio, there is an AI tool for everything.
It is only obvious that AI found its way into analyzing social sentiments. It pits together the power of two of its subfields –
- Natural Language Processing
- Machine Learning
The technology behind sentiment analysis
Any AI-enabled sentiment analysis tool has these two subfields at its core.
NLP converts human language into a language that machines understand. It implements syntactic (understand text structure) and semantic (identify meaning) techniques.
Once the text is processed, machine learning algorithms come into play for classifications. Machine learning algorithms help identify patterns in data and make relevant predictions based on them.
However, you must understand that machine learning algorithms do not rely on explicit instructions. Rather, it learns from its existing data set, i.e., the training data.
In layman’s terms, a machine learning model will classify texts by sentiments based on the text emotions it is trained on. Each emotion must be labeled with corresponding categories and tags.
After seeing a few examples, the model learns to associate a given text with a specific tag. Based on this, it starts predicting tags for unseen content.
Sentiment analysis with AI lets you tag a huge volume of data sets simultaneously and in real-time. Since machine learning algorithms learn over time, your sentiment analysis models will only get smarter.
Social Sentiment Analysis with AI
Social sentiment analysis tools follow the same model. It scouts social channels to identify and classify texts (social messages) into positive or negative. For instance, a social post stating, “I love using this tool” will give positive results in sentiment analysis.
Check out how sentiment analysis work.
Featuring: MonkeyLearn’s Free Sentiment Analysis Tool.
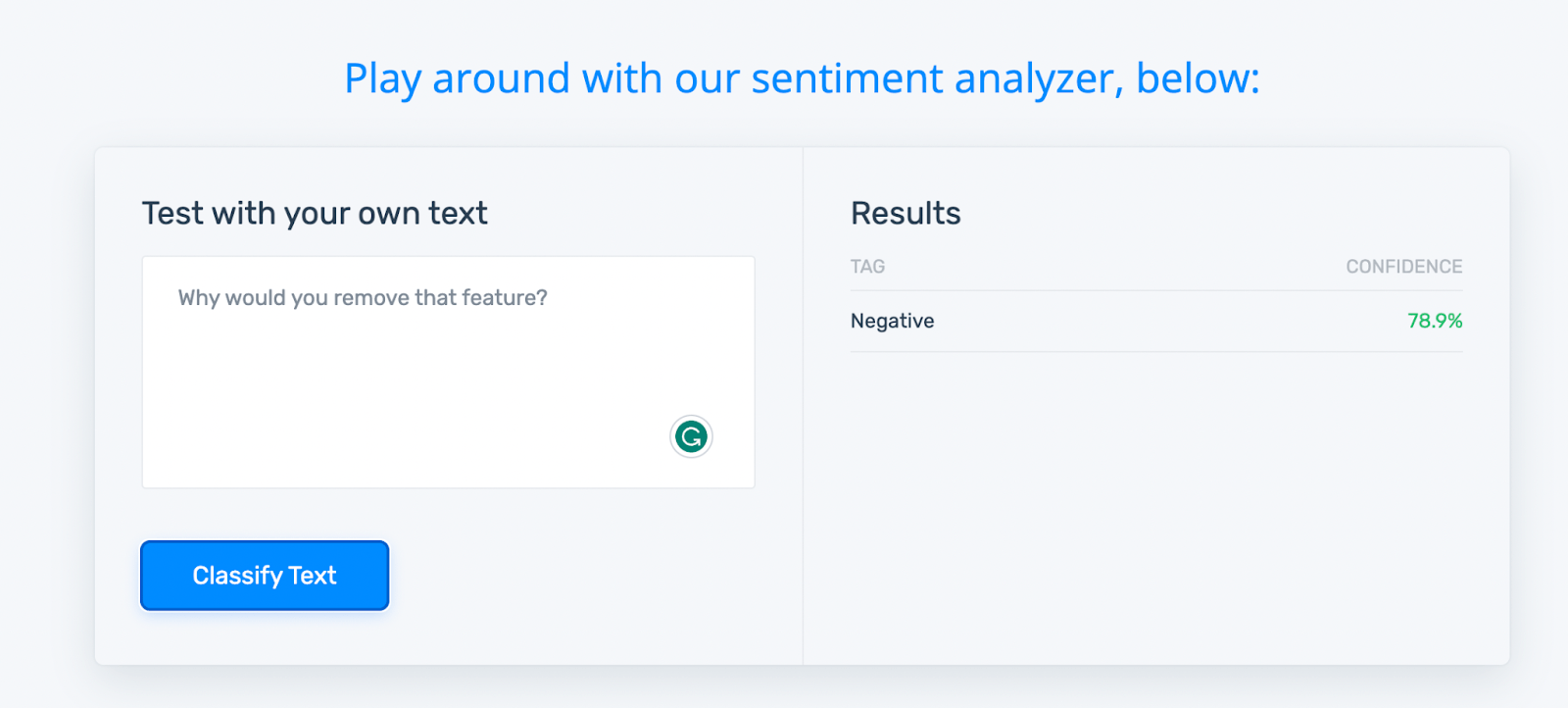
But, is it the same as social listening?
While marketers use these two terms interchangeably, they are different.
Social Listening | Social sentiment analysis |
Social listening captures brand mentions across the web. It is not limited to social channels. Brand mentions can be anywhere – blogs, social channels, forums, communities, etc. It helps answer questions like – Where do people talk about my brand? How do they perceive my brand? How does my brand perform against my competitors? Who can be my key influencers? |
Social analytics provide actionable insights. It analyzes brand performance on social media at a deeper level and helps you understand the impact of your social efforts. It helps answer questions like: How effectively users are engaging on social media? How many people can see your brand? What are the user demographics and interests? |
Social sentiment analysis can be tagged as a subset of social listening. Once you discover your brand mentions, the next step is to analyze the sentiment across those mentions.
This allows brands to detect emergencies and take appropriate action before it is late. After all, more social mentions are not always equal to positive mentions.
For instance, the eCommerce giant was in hot soup in 2019 for apparently hurting Hindu sentiments when it started showcasing products like doormats, slippers, and toilet seat covers with pictures of Hindu gods and goddesses. A Twitter user posted images of the same and was backed by thousands of people urging the brand to remove the entire catalog.
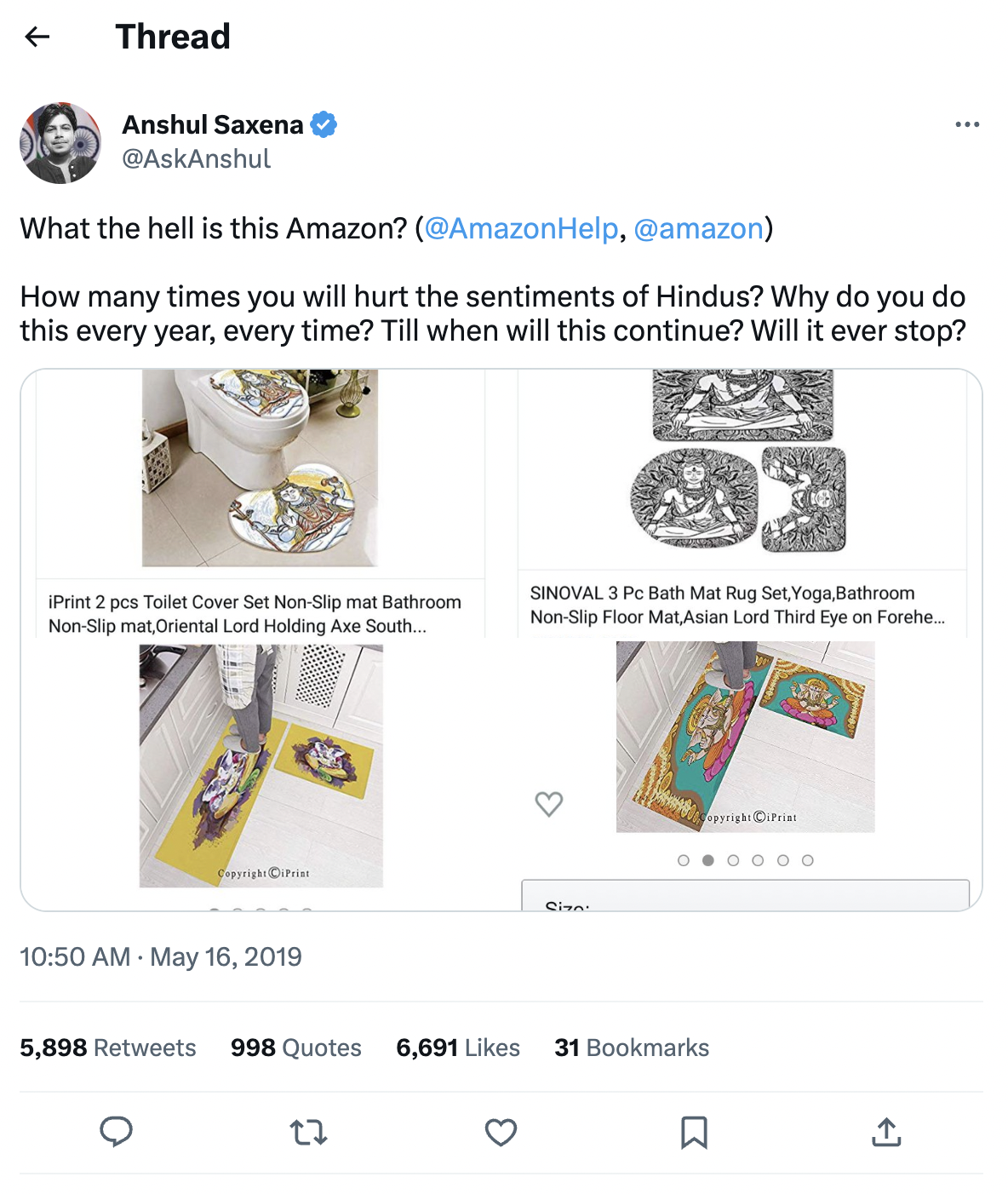
The aggression was severe, to the point where users threatened to stop using the app or delete the app, altogether! And why not. It was the second time Amazon made the same mistake.
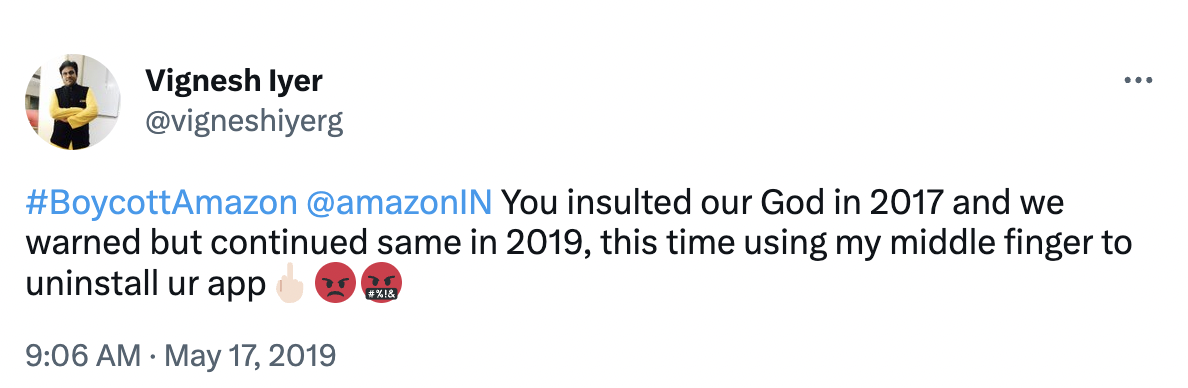
As a result, Amazon clarified that the said product catalogs are removed and that all sellers will adhere to the selling guidelines. If sellers do not adhere to the selling guidelines, their products will be removed from the eCommerce portal.
Owing up social backlash with utmost honesty and rectifying mistakes – brands can do it right if they successfully listen to their users and analyze the sentiment around the chatter.
Types of Social Sentiment Analysis
Opinions (or sentiments) on social media are wide-ranged. They can be extremely positive or negative, somewhat positive or negative, or absolutely neutral. This is also termed as polarity-driven analysis.
Here’s how you can grade emotions from the tone of the text.
User opinion | Sentiment analysis |
I am so stocked to see this feature live. Can’t wait to try it out | Extreme positive |
Why would you remove the icon @xyz brand? | Somewhat negative |
I am never coming back to this website ever, ever again. Pathetic customer service. | Extreme negative |
Not sure if this feature will help marketers in the long run | Neutral |
I love buying from @xyz but this time you’ve really goofed up | Somewhat negative |
Sentiment analysis models
Grading sentiments as positive, negative, or neutral is the first level. To implement AI-enabled social sentiment analysis, you must go beyond polarity.
Some advanced and popular types of sentiment analysis include:
- Emotion analysis
Emotion-driven analysis helps you dig deeper into user emotions like anger, happiness, or frustration around your product/brand. In most cases, sentiment analysis tools use lexicons i.e. list of words that conveys emotions, or resort to advanced machine learning algorithms.
The downside of using lexicons is that the correct emotion is often not captured. Lexicons contain words that specify a certain kind of emotion. For instance, words like bad or kill often resonates with anger. However, a social opinion stating this feature is killing it indicates happiness.
For instance, look at this tweet with all the bad words, but the tweet’s emotion is positive and happy. In such cases, the emotion analysis can go wrong unless your sentiment analysis also considers the emojis.
In that case, the algorithm will predict the emotion correctly.
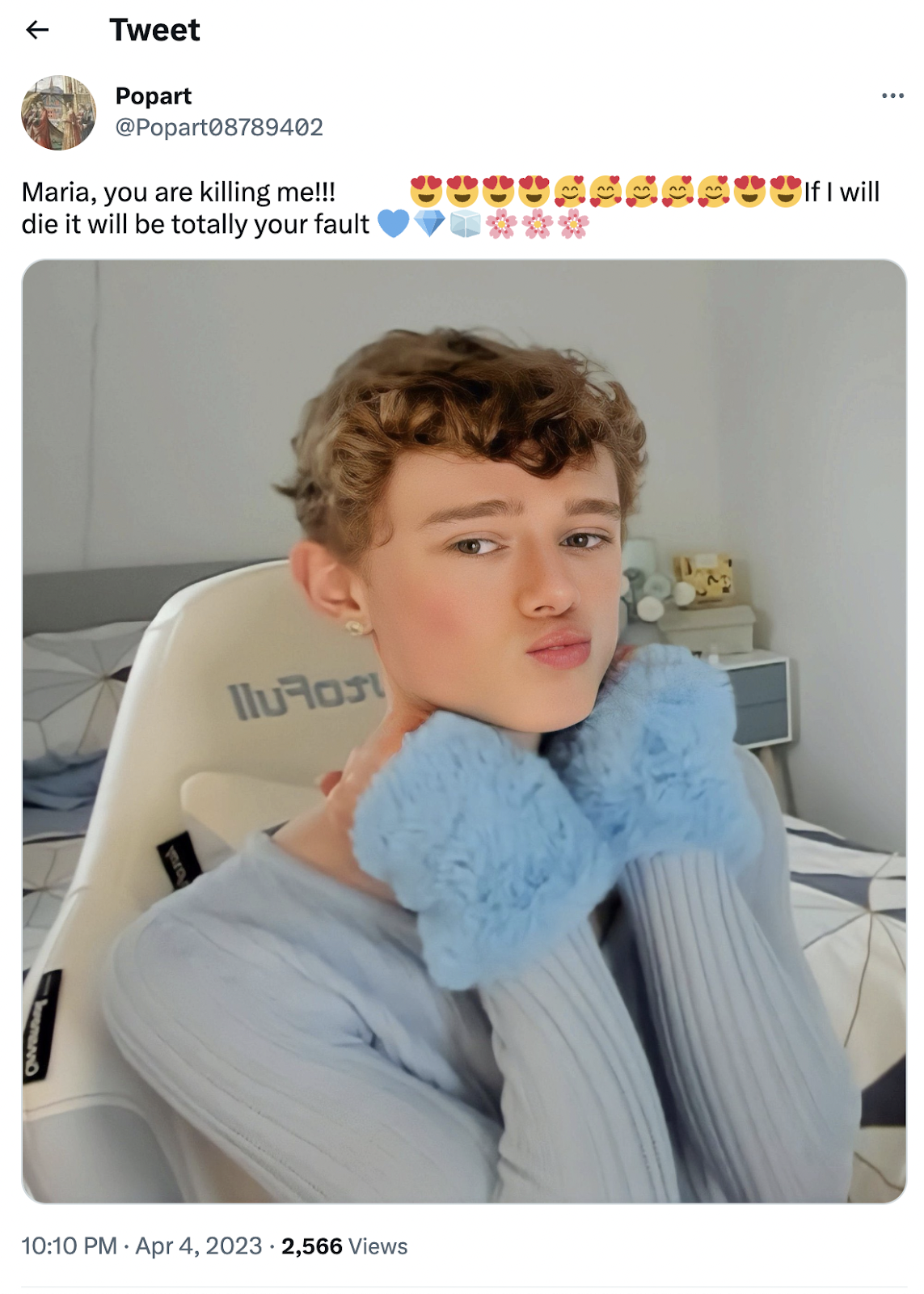
- Aspect-based analysis
Aspect-based analysis helps brands understand what exact feature or part of ad users tag as good, bad, or ugly!
For instance, This filter does not look good on nigh-mode pictures instantly tells a brand that the opinion is solely about the said filter. The brand can improve the filter or launch a new one for the camera’s night mode. Either way, aspect-based analysis helps dig deep into what users want and what they do not.
- Multilingual sentiments
This involves a lot of pre-processing and resources. You will need lexicons (available online), noise, and translation algorithms (you must build them) to detect the sentiment.
Multilingual sentiment analysis works for brands with users from varied ethnicities, cultures, and demographics.
Social sentiment analysis to Understand Customers
There is no other alternative for brands but to listen to and understand their end-users. As more and more users become aware of the power of social media and brand positioning, brands must leverage social monitoring and sentiment analysis.
Here’s how you can use sentiment analysis to your benefit.
1. Spot opportunities to improve user experience
When you start monitoring brand mentions across social channels (and the web), you monitor compliments and complaints. This gives you a detailed insight into what your users expect from you. While compliments show that your product/services are built in the right direction, complaints show the gap that you might be missing otherwise.
For instance, a complaint need not be aggressive or extremely negative. It can also be around a missing feature or service you have not yet introduced. Monitoring such mentions and engaging with users can help you identify what your users seek. If you find most users voting for that missing feature, you know it’s time to get it done.
One brand that I absolutely love using is Canva, not only because the templates and design possibilities help marketers like me design pro images – but also because this brand listens and responds.
Check this tweet from one of its users and how Canva handled it.
There are many instances when you feel like a feature is missing and validate the same via social media. Social posts like these are also part of sentiment analysis. The score may not be as high as a proper negative review, but it will still be graded as negative.
Pro-tip to handle such mentions: Own up to the gap and be empathetic. This lets the user know you’ve noted the issue and will work around it. It builds trust and also improves user experience. When a user knows he/she is heard, that’s all that matters to build a healthy brand reputation.
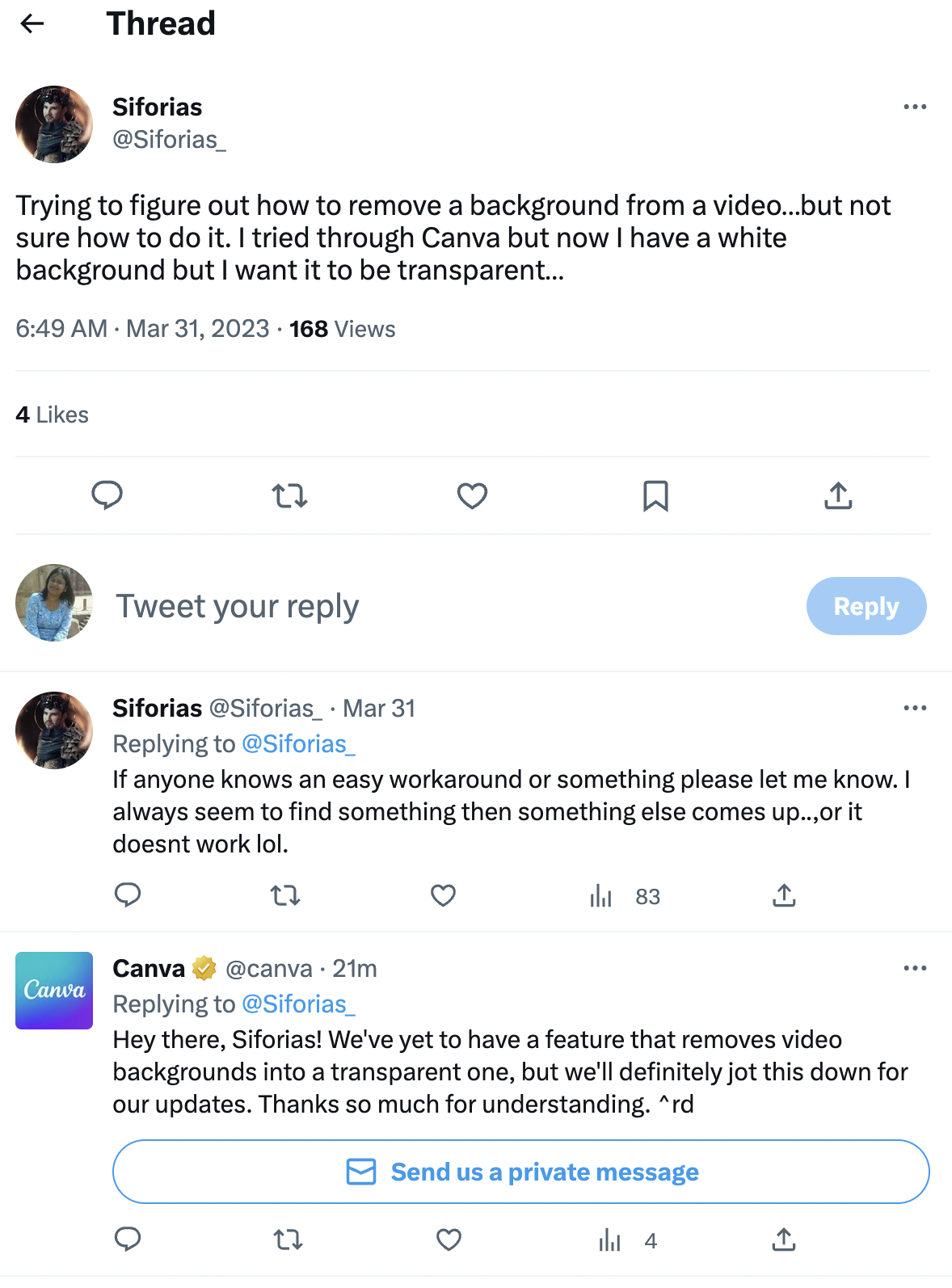
2. Reduce customer wait time
Sentiment analysis tools will show you sentiments over time, like days, weeks, and months. Simultaneously, it will also tag social posts as positive, negative, and neutral for you to take quick action.
For instance, I used a free sentiment analyzer tool to review the sentiment score for a random post.
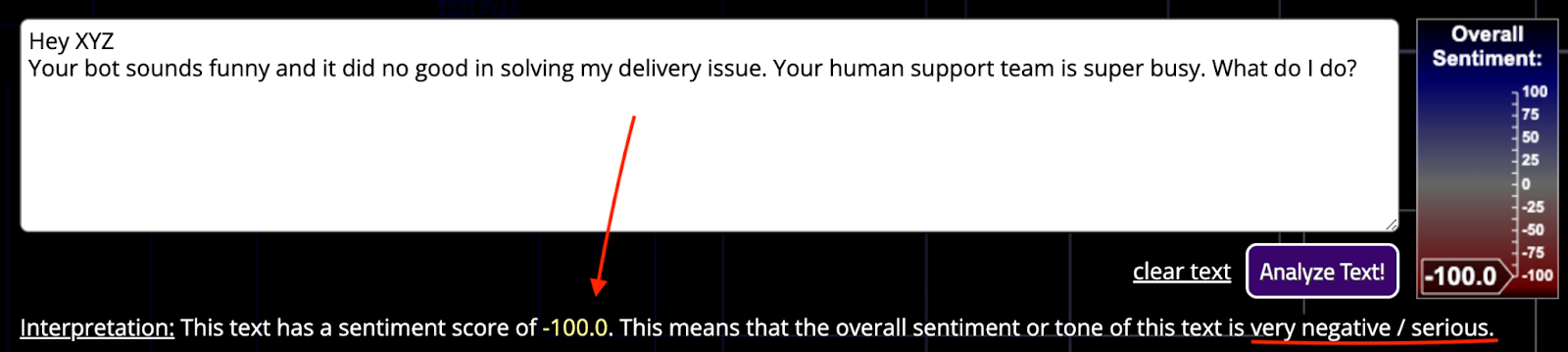
The tool analyzed the text and scored -100, indicating extremely negative and serious issues. The post uses a sarcastic tone to complain, but the tool accurately captures the negative sentiment.
Similarly, I used one of the genuine tweets from Canva’s Twitter channel and ran an analysis on the same –
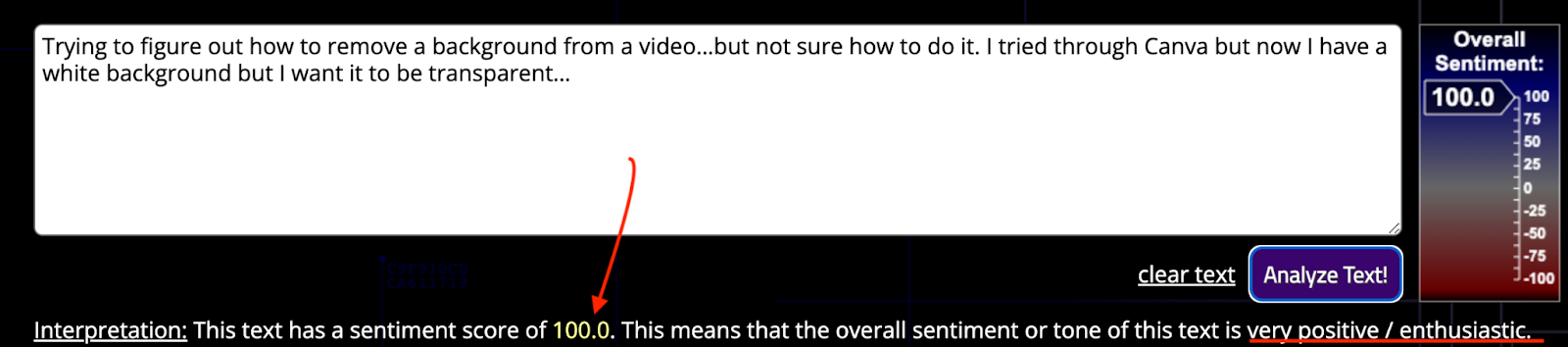
While this tweet is regarding a missing feature in the product, the sentiment is still positive and generates a 100 score on the sentiment scorecard. It contains lexicons like ‘not sure’, which can easily get tagged as negative sentiments. However, looking at the context, the tool marks it as positive.
In both cases, you must be quick to respond.
- Responding to a positive or neutral social post will help you build better relationships with your users, along with instant gratification.
- Responding to an issue or complaint on social media posts with either a solution or a process/resource to resolve the issue quickly can help you build user retention.
No one wants to wait endlessly. Forget endless; users hate to wait beyond an hour. Research shows users expect a reply within 24 hours. Your users will start rethinking your brand within seconds of encountering an issue. So, the quicker you are, the better it is.
For instance, Apple’s customer support is always prompt in its reply. While it does not fully resolve the issue immediately, the response time is less than a minute which is a breather for any users stuck with an issue. The response contains either some general steps to resolve the issue or a quick message to DM them the issue using the send us a private message CTA included in the response. [Tweet]
What I love about this social handle is that every response (although these are automated) has customization. For example, the user complains about iMessage, so the response includes the ‘iMessage’ term to give it a personal touch.
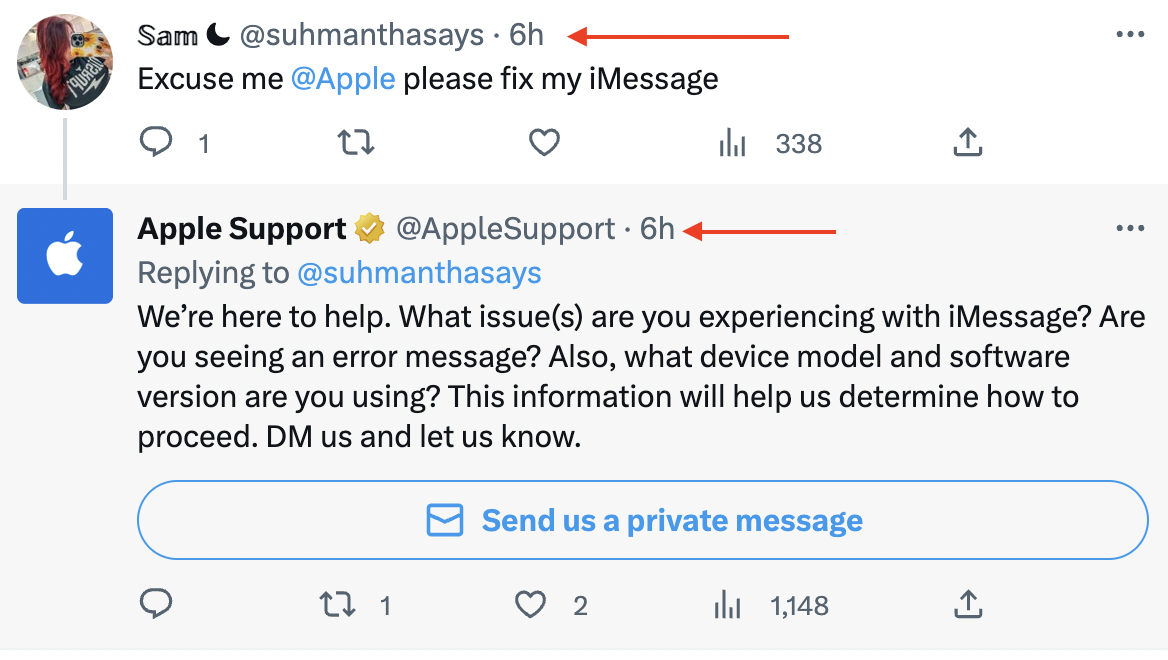
Pro-tip to reduce wait time: The best way to reduce wait time is by automating tas tagging for incoming support tickets.
You can train your customer support team to use sentiment analysis to tag incoming tickets and automatically put negative tickets at the top of the queue.
Simultaneously, you can create rules to automatically get negative tickets assigned to the most experienced representative to guarantee the best attention.
3. Define Neutral and Emojis for accurate analysis
Defining positive and negative is easier than defining neutral. It is hard to train your machine-learning model to detect neutral tags.
How you perceive neutral plays an important role in training your sentiment analysis model. Each tagging data will require tagging criteria so that a good definition can go a long way. Here’s how you can do this:
- Classify all objective texts as neutral, especially those that do not have explicit sentiments.
- If your data is still pre-processed, you can tag it neutral since the irrelevant data is yet to be filtered. But do it with caution. Sometimes, it may add more noise and hamper the performance.
- Texts like “I wish it could do XYZ” are usually neutral. However, you may find it harder to categorize wish texts like “I wish xyz did better than abc”.
Next comes categorizing emojis. Emojis are of two types:
- Western emojis with two characters
- Easter emojis with longer combinations of characters of vertical nature
Emojis play a pivotal role in determining the sentiment of the texts, especially in tweets.
In this case, you must be careful of character and word levels when performing sentiment analysis.
Pro Tip: Consider pre-processing social media content, transforming emojis into tokens, and whitelisting them.
Twitter offers instant gratification, which makes it the most accurate blueprint of user opinion. Monitoring brand mentions and competitor mentions can help in understanding the ‘emotion’ of users, how they feel about a product or a particular feature, and what can trigger them incorrectly.
Twitter sentiment analysis can be done using programming languages like R and Python. Below are the steps for Twitter data sentiment analysis.
1. Extract and collect data
To mine data from Twitter, you can use Twitter APIs like Tweepy and TextBlob. Data extraction is the crux of the process because all other steps directly depend on this.
Ensure that your Twitter data contains the observations you want to analyze. You will find two types of tweets:
- Latest tweets under real-time hashtags, keywords, or topics.
- Historical tweets posted in the past and their responses in different timelines.
2. Pre-process sentiment analysis dataset
Tweets are mostly unstructured data and require extensive pre-processing before using them as training data for the sentiment analysis model. Data reduction is one way of preparing data for mining.
This includes:
- Cleaning all the noise from the data
- Deleting duplicate or meaningless tweets [eg, tweets shorter than three characters]
- Formatting improvements and concatenation
- Preparing custom data to perform testing on the model
Clean and good data facilitates precise outcomes, i.e. more accurate predictions.
3. Build an ML model for sentiment analysis
The model building depends on the problem statement, requirements, and use cases. However, it has 5 mandates –
- Create the base model type as a Classifier to categorize and define tags
- Under the model, create a sentiment analysis project for the classification
- Import the pre-processed data as the training dataset for model
- Train the model by tagging each tweet as positive, negative, or neutral. After a few manual tagging, the models learn to do the tagging with maximum accuracy.
- Test the model performance and preciseness of predictions. The larger dataset you use, the easier it is for the model to learn the tagging.
Tip for maximum accuracy: Define tags more explicitly in the training data and crosscheck for false positives and negatives using various test cases.
4. Analyse the data for sentiment analysis
Integrate Twitter data with the tweet sentiment analysis model. There are pre-built APIs that require tokenization and API calls to complete the integration process.
Examples: Inference API by Huggice Face, Lexanalytics, Brandwatch, Rosette, Social Mention, and more.
5. Use data visualization to demonstrate your findings
Data visualization illustrates any sentiment analysis report the best. It is easy to understand, interactive, and dynamic. In addition, they work on business intelligence frameworks and render impeccable data visuals.
Examples: Power BI, Google Data Studio, Tableau, Klipfolio, etc.
Closing statement
Social sentiment analysis has proven benefits like –
- Large-scale data sorting
- Real-time data analysis
- Improved user experience
- Detailed competitor analysis
It can be applied to innumerable aspects of your business – be it brand monitoring, product analytics, market research, and customer service. Social sentiment analysis helps brands work fast and work smartly. It works towards listening to users more accurately and making the end-user experience worthwhile.
Sentiment analysis is already mandated for brands who want to build products or design services catered to humans. It gives a deeper insight into user intent, emotions, and engagements for teams to work more effectively.
Have you started analyzing your users’ emotions across social channels?
Featured image by Luis Quintero on Pexels