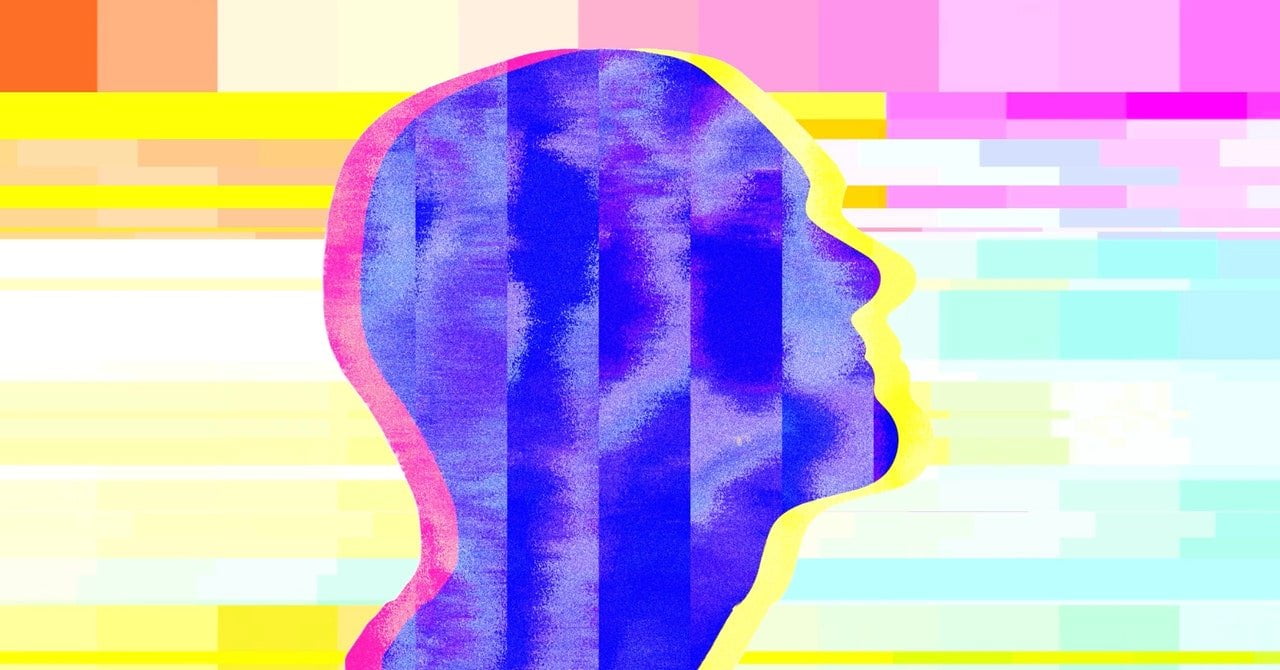
If 2022 was the year the generative AI boom started, 2023 was the year of the generative AI panic. Just over 12 months since OpenAI released ChatGPT and set a record for the fastest-growing consumer product, it appears to have also helped set a record for fastest government intervention in a new technology. The US Federal Elections Commission is looking into deceptive campaign ads, Congress is calling for oversight into how AI companies develop and label training data for their algorithms, and the European Union passed its new AI Act with last-minute tweaks to respond to generative AI.
But for all the novelty and speed, generative AI’s problems are also painfully familiar. OpenAI and its rivals racing to launch new AI models are facing problems that have dogged social platforms, that earlier era-shaping new technology, for nearly two decades. Companies like Meta never did get the upper hand over mis- and disinformation, sketchy labor practices, and nonconsensual pornography, to name just a few of their unintended consequences. Now those issues are gaining a challenging new life, with an AI twist.
“These are completely predictable problems,” says Hany Farid, a professor at the UC Berkeley School of Information, of the headaches faced by OpenAI and others. “I think they were preventable.”
In some cases, generative AI companies are directly built on problematic infrastructure put in place by social media companies. Facebook and others came to rely on low-paid, outsourced content moderation workers—often in the Global South—to keep content like hate speech or imagery with nudity or violence at bay.
That same workforce is now being tapped to help train generative AI models, often with similarly low pay and difficult working conditions. Because outsourcing puts crucial functions of a social platform or AI company administratively at arms length from its headquarters, and often on another continent, researchers and regulators can struggle to get the full picture of how an AI system or social network is being built and governed.
Outsourcing can also obscure where the true intelligence inside a product really lies. When a piece of content disappears, was it taken down by an algorithm or one of the many thousands of human moderators? When a customer service chatbot helps out a customer, how much credit is due to AI and how much to the worker in an overheated outsourcing hub?
There are also similarities in how AI companies and social platforms respond to criticism of their ill or unintended effects. AI companies talk about putting “safeguards” and “acceptable use” policies in place on certain generative AI models, just as platforms have their terms of service around what content is and is not allowed. As with the rules of social networks, AI policies and protections have proven relatively easy to circumvent.
Shortly after Google released its Bard chatbot this year, researchers found major holes in its controls; in tests they were able to generate misinformation about Covid-19 and the war in Ukraine. In response to WIRED’s reporting on that problem, a Google spokesperson called Bard “an early experiment that can sometimes give inaccurate or inappropriate information” and claimed the company would take action against problematic content.
It’s unclear whether chatbot providers can make their creations reliable enough to escape the reactive cycle seen at social platforms, which constantly but unreliably police the fire hose of new, problematic content. Although companies like Google, Amazon, and OpenAI have committed to some solutions, like adding digital “watermarks” to AI-generated videos and photos, experts have noted that these measures too are easy to circumvent and are unlikely to function as long-term solutions.
Farid predicts that just as social media platforms amplified the power of individuals to produce and share information—whether or not it was accurate or shared in good faith—generative AI will take that capacity to another level. “We are seeing a lot more disinformation,” he says. “People are now using that generated AI to create videos of candidates and politicians and news anchors and CEOs saying things they never said.”
Farid says that not only does generative AI make producing mis- and disinformation faster, cheaper, and easier, it also undermines the veracity of real media and information. Just as Donald Trump sought to neutralize unfavorable coverage by calling it “fake news,” earlier this year an Indian politician alleged that leaked audio implicating him in a corruption scandal was fake (it wasn’t).
In response to concerns that fake videos of US political candidates could distort 2024 election campaigns, Meta and YouTube released policies requiring AI-generated political advertisements to be clearly labeled. But like watermarking generated images and video, the policy doesn’t cover a variety of other ways fake media can be created and shared.
Despite what appears to be a worsening outlook, platforms have begun to cut back on the resources and teams needed to detect harmful content, says Sam Gregory, program director at the nonprofit Witness, which helps people use technology to promote human rights. Major tech companies have laid off tens of thousands of workers over the past year. “We’ve seen a lot of cutbacks in trust and safety teams and fact-checking programs at the same time, and these are adding a very unstable wild card to the mix,” he says. “You’re reducing the capacity, both within companies and within civil society, to be able to pay attention to how that’s used deceptively or maliciously.”
The unintended consequences of social platforms came to be associated with a slogan once popular with Facebook CEO Mark Zuckerberg: “Move fast and break things.” Facebook later retired that motto, but as AI companies jockey for supremacy with generative algorithms, Gregory says he is seeing the same reckless approach now.
“There’s a sense of release after release without much consideration,” he says, noting that, like many large platforms, there is an opacity to how these products are being built, trained, tested, and deployed.
Although the US Congress and regulators around the globe seem determined to react to generative AI less sluggishly than they did to social media, Farid says regulation is lagging far behind AI development. That means there’s no incentive for the new crop of generative-AI-focused companies to slow down out of concern for penalties.
“Regulators have no idea what they’re doing, and the companies move so fast that nobody knows how to keep up,” he says. It points to a lesson, he says, not about technology, but about society. “The tech companies realize they can make a lot of money if they privatize the profits and socialize the cost. It’s not the technology that’s terrifying, what’s terrifying is capitalism.”
What Generative AI Failed to Learn from Web 2.0
Generative Artificial Intelligence (AI) has made significant strides in recent years, with impressive advancements in natural language processing, image generation, and even music composition. However, despite these achievements, there are lessons that generative AI has failed to learn from the Web 2.0 era. In this article, we will explore some of these missed opportunities and discuss their potential impact on the future development of AI.
One of the key aspects that generative AI has failed to grasp from Web 2.0 is the importance of user-generated content and collaboration. Web 2.0 revolutionized the internet by enabling users to actively participate in content creation, sharing, and collaboration. Platforms like Wikipedia, YouTube, and social media sites empowered individuals to contribute their knowledge, creativity, and opinions to a global audience.
Generative AI, on the other hand, still heavily relies on pre-existing data sets and lacks the ability to actively engage with users for content creation. While AI models can generate impressive outputs based on training data, they often struggle to adapt to new information or incorporate user feedback effectively. This limitation hampers the potential of generative AI to evolve and improve over time through collective intelligence.
Another missed opportunity for generative AI is the concept of democratization. Web 2.0 democratized access to information, allowing anyone with an internet connection to contribute, share, and access knowledge. This democratization led to a more inclusive and diverse online ecosystem.
However, generative AI has not fully embraced this principle. The development and deployment of advanced AI models still largely remain in the hands of a few tech giants and research institutions due to the complexity and resources required. This lack of democratization limits the potential of generative AI to benefit society as a whole and hinders its progress towards more inclusive and equitable applications.
Furthermore, generative AI has yet to fully understand the importance of transparency and explainability. Web 2.0 emphasized the need for transparency in algorithms and decision-making processes. Users were able to understand how their data was being used, and platforms were held accountable for their actions.
In contrast, generative AI often operates as a black box, making it challenging to understand how it arrives at its outputs. This lack of transparency raises concerns about bias, ethics, and accountability. Without clear explanations, it becomes difficult to trust and responsibly deploy generative AI systems in critical domains such as healthcare, finance, or law.
Lastly, generative AI has not fully embraced the power of community-driven innovation. Web 2.0 thrived on the open-source movement, where developers collaborated and shared their work freely. This collective effort led to rapid advancements and breakthroughs in various fields.
Generative AI, however, still faces challenges in fostering a vibrant open-source community. While there are initiatives to share pre-trained models and code, the collaborative spirit that drove Web 2.0 is yet to be fully realized in the AI community. Encouraging open collaboration and knowledge sharing could accelerate the development of generative AI and lead to more robust and reliable models.
In conclusion, while generative AI has achieved remarkable progress, it has failed to learn some crucial lessons from the Web 2.0 era. Embracing user-generated content, democratization, transparency, and community-driven innovation could unlock the full potential of generative AI and ensure its responsible and inclusive development. By incorporating these principles into AI systems, we can create a future where AI truly benefits society as a whole.