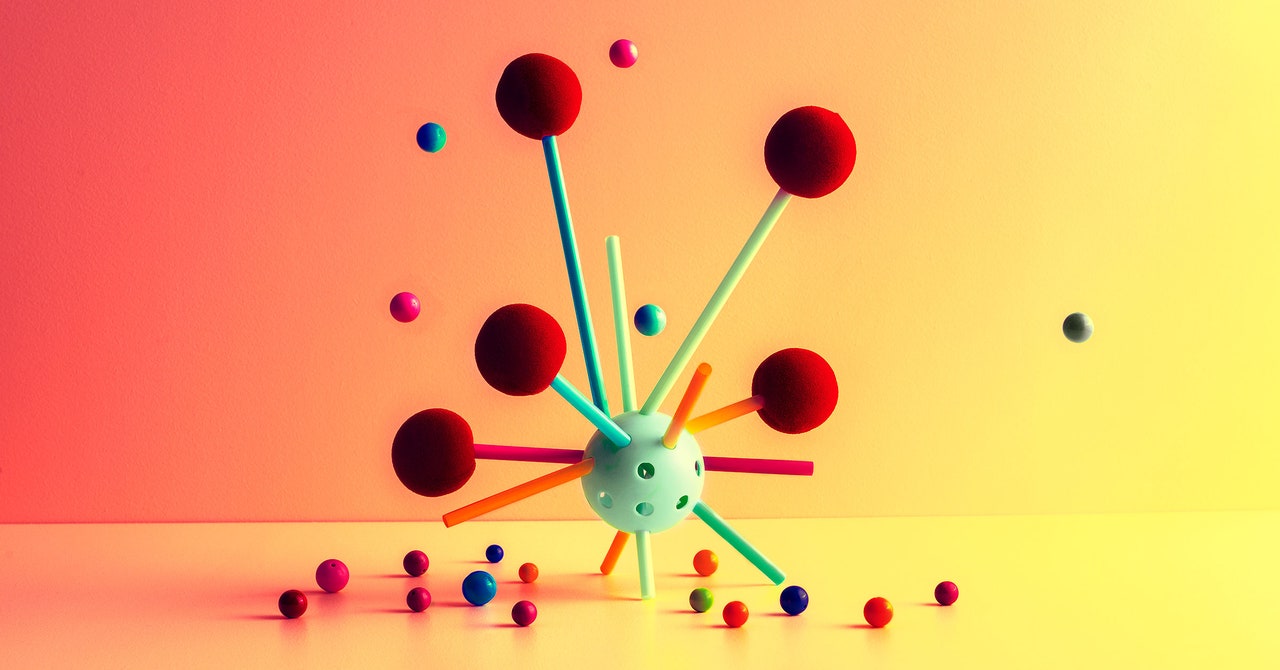
Google spent much of the past year hustling to build its Gemini chatbot to counter ChatGPT, pitching it as a multifunctional AI assistant that can help with work tasks or the digital chores of personal life. More quietly, the company has been working to enhance a more specialized artificial intelligence tool that is already a must-have for some scientists.
AlphaFold, software developed by Google’s DeepMind AI unit to predict the 3D structure of proteins, has received a significant upgrade. It can now model other molecules of biological importance, including DNA, and the interactions between antibodies produced by the immune system and the molecules of disease organisms. DeepMind added those new capabilities to AlphaFold 3 in part through borrowing techniques from AI image generators.
“This is a big advance for us,” Demis Hassabis, CEO of Google DeepMind, told WIRED ahead of Wednesday’s publication of a paper on AlphaFold 3 in the science journal Nature. “This is exactly what you need for drug discovery: You need to see how a small molecule is going to bind to a drug, how strongly, and also what else it might bind to.”
AlphaFold 3 can model large molecules such as DNA and RNA, which carry genetic code, but also much smaller entities, including metal ions. It can predict with high accuracy how these different molecules will interact with one another, Google’s research paper claims.
The software was developed by Google DeepMind and Isomorphic labs, a sibling company under parent Alphabet working on AI for biotech that is also led by Hassabis. In January, Isomorphic Labs announced that it would work with Eli Lilly and Novartis on drug development.
AlphaFold 3 will be made available via the cloud for outside researchers to access for free, but DeepMind is not releasing the software as open source the way it did for earlier versions of AlphaFold. John Jumper, who leads the Google DeepMind team working on the software, says it could help provide a deeper understanding of how proteins interact and work with DNA inside the body. “How do proteins respond to DNA damage; how do they find, repair it?” Jumper says. “We can start to answer these questions.”
Understanding protein structures used to require painstaking work using electron microscopes and a technique called x-ray crystallography. Several years ago, academic research groups began testing whether deep learning, the technique at the heart of many recent AI advances, could predict the shape of proteins simply from their constituent amino acids, by learning from structures that had been experimentally verified.
In 2018, Google DeepMind revealed it was working on AI software called AlphaFold to accurately predict the shape of proteins. In 2020, AlphaFold 2 produced results accurate enough to set off a storm of excitement in molecular biology. A year later, the company released an open source version of AlphaFold for anyone to use, along with 350,000 predicted protein structures, including for almost every protein known to exist in the human body. In 2022 the company released more than 2 million protein structures.
The latest AlphaFold’s ability to model different proteins was improved in part through an algorithm called a diffusion model that helps AI image generators like Dall-E and Midjourney create weird and sometimes photo-real imagery. The diffusion model inside AlphaFold 3 sharpens the molecular structures the software generates. The diffusion model is able to generate plausible protein structures based on patterns it picked up from analyzing a collection of verified protein structures, much as an image generator learns from real photographs how to render realistic-looking snapshots.
AlphaFold 3 is not perfect, though, and offers a color-coded confidence scale for its predictions. Areas of a protein structure colored blue indicate high confidence, while red areas show less certainty.
David Baker, a professor at the University of Washington who leads a group working on techniques for protein design, has competed with AlphaFold. In 2021, before DeepMind open sourced its creation, his team released an independent protein-structure prediction inspired by AlphaFold. His own lab recently released a diffusion model to help model a wider range of molecular structures, but he concedes that AlphaFold 3 is more capable. “The structure prediction performance of AlphaFold 3 is very impressive,” Baker says.
Baker adds that it is a shame that the source code for AlphaFold 3 has not been released to the scientific community.
Hassabis, who leads all of Alphabet’s AI initiatives, has long taken a special interest in the potential for AI to accelerate scientific research. But he says the latest techniques being developed for AlphaFold, a highly specialized AI system, could prove useful for building more general systems that aim to exceed human capabilities on many dimensions.
If AI programs like Google’s Gemini become a lot more capable over the next decade, he says, “you could imagine them using things like AlphaFold as tools, to achieve some other goal.”
Google DeepMind, the renowned artificial intelligence (AI) research lab, has made another groundbreaking achievement by developing a new AI system capable of modeling DNA structures in addition to protein structures. This development marks a significant step forward in understanding the fundamental building blocks of life and has the potential to revolutionize various fields, including medicine, genetics, and bioengineering.
Proteins and DNA are vital components of all living organisms. Proteins play a crucial role in various biological processes, such as cell signaling, enzyme catalysis, and immune responses. On the other hand, DNA contains the genetic instructions necessary for the development, functioning, and reproduction of all known living organisms. Understanding the structure and function of proteins and DNA is essential for deciphering the complexities of life itself.
DeepMind’s AI system, known as AlphaFold, gained international recognition in 2020 when it won the Critical Assessment of Structure Prediction (CASP) competition. CASP is a biennial event where researchers from around the world compete to predict the 3D structure of proteins based solely on their amino acid sequences. AlphaFold’s victory was a significant breakthrough, as accurately predicting protein structures had long been considered one of the most challenging problems in biology.
Building upon this success, DeepMind expanded AlphaFold’s capabilities to include modeling DNA structures. The AI system was trained on a vast dataset of known DNA structures, allowing it to learn the complex rules governing DNA folding. By analyzing the sequence of nucleotides that make up a DNA molecule, AlphaFold can predict its 3D structure with remarkable accuracy.
The ability to accurately model DNA structures has immense implications for various scientific fields. One of the most significant applications is in understanding genetic diseases. Many genetic disorders are caused by mutations that disrupt the normal structure and function of DNA. By accurately predicting the structure of mutated DNA, scientists can gain insights into how these mutations lead to disease and potentially develop targeted therapies.
Furthermore, AlphaFold’s DNA modeling capabilities can aid in the field of synthetic biology. Synthetic biologists engineer organisms to produce valuable compounds or perform specific functions. Understanding the 3D structure of DNA is crucial for designing synthetic genetic circuits that can control gene expression and regulate cellular processes. AlphaFold’s predictions can guide scientists in designing more efficient and reliable genetic circuits, accelerating advancements in bioengineering.
Another area that stands to benefit from AlphaFold’s new capabilities is drug discovery. Proteins are the primary targets for most drugs, and understanding their structure is crucial for designing effective therapeutic interventions. However, proteins often interact with DNA, and their structures can be influenced by DNA binding. By accurately modeling both protein and DNA structures, scientists can gain a more comprehensive understanding of these interactions, leading to the development of more precise and potent drugs.
While DeepMind’s achievement in modeling DNA structures is undoubtedly groundbreaking, there are still challenges to overcome. The AI system’s predictions need to be experimentally validated to ensure their accuracy and reliability. Additionally, the current version of AlphaFold focuses on predicting the static structures of DNA and proteins, but dynamic changes in structure are also essential for understanding their functions fully.
Nonetheless, DeepMind’s progress in expanding AlphaFold’s capabilities to model DNA structures represents a significant leap forward in our understanding of the fundamental components of life. With further advancements and collaborations with the scientific community, this technology has the potential to revolutionize various fields, unlocking new insights into genetics, medicine, and bioengineering.